【PCL】sample_consensus 模块—— Random Sample Consensus model(随机样本一致性模型,RANSAC)
1、随机样本一致性模型(RANSAC)简介
在本教程中,我们将学习如何使用带有平面模型的随机样本一致性(RANSAC)来获取适合该模型的点云。
1.1理论背景
RANSAC 是“随机样本一致性”(RANdom SAmple Consensus)的缩写,它是一种迭代方法,用于从包含异常值的数据集中估计数学模型的参数。该算法由 Fischler 和 Bolles 于 1981 年提出。RANSAC 算法假设我们观察到的数据由内点和异常值组成。内点可以通过具有特定参数值的模型来解释,而异常值在任何情况下都不适合该模型。另一个必要的假设是,存在一种可以从数据中最佳估计所选模型参数的程序。
RANSAC 算法的输入是一组观测数据值、一个可以解释或拟合观测值的参数化模型以及一些置信参数。
RANSAC 通过迭代选择原始数据的随机子集来实现其目标。这些数据是假设的内点,然后通过以下步骤测试该假设:
- 将模型拟合到假设的内点,即从内点重建模型的所有自由参数。
- 然后,所有其他数据都针对拟合模型进行测试,如果某个点与估计模型拟合良好,则也将其视为假设的内点。
- 如果足够多的点被分类为假设的内点,则估计的模型是合理的。
- 从所有假设的内点重新估计模型,因为它仅从初始的假设内点集中估计。
- 最后,通过估计内点相对于模型的误差来评估模型。
此过程重复固定次数,每次生成一个由于分类为内点的点太少而被拒绝的模型,或者生成一个带有相应误差度量的改进模型。在后一种情况下,如果其误差低于上次保存的模型,则保留改进的模型。
RANSAC 的一个优点是它能够对模型参数进行鲁棒估计,即即使数据集中存在大量异常值,它也可以高精度地估计参数。RANSAC 的一个缺点是计算这些参数所需的时间没有上限。当计算的迭代次数有限时,获得的解决方案可能不是最优的,甚至可能不是一个很好地拟合数据的解决方案。通过这种方式,RANSAC 提供了一种权衡;通过计算更多的迭代次数,生成合理模型的概率会增加。RANSAC 的另一个缺点是它需要设置特定问题的阈值。
RANSAC 只能估计一个特定数据集的一个模型。对于任何单一模型方法,当存在两个(或更多)模型时,RANSAC 可能无法找到任何一个。
1.2图像示例
左侧和右侧的图像展示了 RANSAC 算法在二维数据集上的简单应用。左侧的图像是包含内点和异常值的数据集的视觉表示。右侧的图像以红色显示所有异常值,并以蓝色显示内点。蓝线是 RANSAC 工作的结果。在这种情况下,我们试图拟合数据的模型是一条线,看起来它非常适合我们的数据。
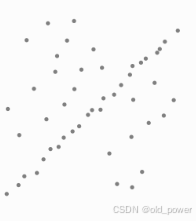
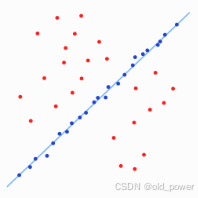
2、代码实现
2.1 RandomSampleConsensusModel.cpp
#include <iostream>
#include <thread>
#include <pcl/console/parse.h>
#include <pcl/point_cloud.h> // for PointCloud
#include <pcl/common/io.h> // for copyPointCloud
#include <pcl/point_types.h>
#include <pcl/sample_consensus/ransac.h>
#include <pcl/sample_consensus/sac_model_plane.h>
#include <pcl/sample_consensus/sac_model_sphere.h>
#include <pcl/visualization/pcl_visualizer.h>
using namespace std::chrono_literals;
pcl::visualization::PCLVisualizer::Ptr
simpleVis (pcl::PointCloud<pcl::PointXYZ>::ConstPtr cloud)
{
// --------------------------------------------
// -----Open 3D viewer and add point cloud-----
// --------------------------------------------
pcl::visualization::PCLVisualizer::Ptr viewer (new pcl::visualization::PCLVisualizer ("3D Viewer"));
viewer->setBackgroundColor (0, 0, 0);
viewer->addPointCloud<pcl::PointXYZ> (cloud, "sample cloud");
viewer->setPointCloudRenderingProperties (pcl::visualization::PCL_VISUALIZER_POINT_SIZE, 3, "sample cloud");
//viewer->addCoordinateSystem (1.0, "global");
viewer->initCameraParameters ();
return (viewer);
}
int
main(int argc, char** argv)
{
// initialize PointClouds
pcl::PointCloud<pcl::PointXYZ>::Ptr cloud (new pcl::PointCloud<pcl::PointXYZ>);
pcl::PointCloud<pcl::PointXYZ>::Ptr final (new pcl::PointCloud<pcl::PointXYZ>);
// populate our PointCloud with points
cloud->width = 500;
cloud->height = 1;
cloud->is_dense = false;
cloud->points.resize (cloud->width * cloud->height);
for (pcl::index_t i = 0; i < static_cast<pcl::index_t>(cloud->size ()); ++i)
{
if (pcl::console::find_argument (argc, argv, "-s") >= 0 || pcl::console::find_argument (argc, argv, "-sf") >= 0)
{
(*cloud)[i].x = 1024 * rand () / (RAND_MAX + 1.0);
(*cloud)[i].y = 1024 * rand () / (RAND_MAX + 1.0);
if (i % 5 == 0)
(*cloud)[i].z = 1024 * rand () / (RAND_MAX + 1.0);
else if(i % 2 == 0)
(*cloud)[i].z = sqrt( 1 - ((*cloud)[i].x * (*cloud)[i].x)
- ((*cloud)[i].y * (*cloud)[i].y));
else
(*cloud)[i].z = - sqrt( 1 - ((*cloud)[i].x * (*cloud)[i].x)
- ((*cloud)[i].y * (*cloud)[i].y));
}
else
{
(*cloud)[i].x = 1024 * rand () / (RAND_MAX + 1.0);
(*cloud)[i].y = 1024 * rand () / (RAND_MAX + 1.0);
if( i % 2 == 0)
(*cloud)[i].z = 1024 * rand () / (RAND_MAX + 1.0);
else
(*cloud)[i].z = -1 * ((*cloud)[i].x + (*cloud)[i].y);
}
}
std::vector<int> inliers;
// created RandomSampleConsensus object and compute the appropriated model
pcl::SampleConsensusModelSphere<pcl::PointXYZ>::Ptr
model_s(new pcl::SampleConsensusModelSphere<pcl::PointXYZ> (cloud));
pcl::SampleConsensusModelPlane<pcl::PointXYZ>::Ptr
model_p (new pcl::SampleConsensusModelPlane<pcl::PointXYZ> (cloud));
if(pcl::console::find_argument (argc, argv, "-f") >= 0)
{
pcl::RandomSampleConsensus<pcl::PointXYZ> ransac (model_p);
ransac.setDistanceThreshold (.01);
ransac.computeModel();
ransac.getInliers(inliers);
}
else if (pcl::console::find_argument (argc, argv, "-sf") >= 0 )
{
pcl::RandomSampleConsensus<pcl::PointXYZ> ransac (model_s);
ransac.setDistanceThreshold (.01);
ransac.computeModel();
ransac.getInliers(inliers);
}
// copies all inliers of the model computed to another PointCloud
pcl::copyPointCloud (*cloud, inliers, *final);
// creates the visualization object and adds either our original cloud or all of the inliers
// depending on the command line arguments specified.
pcl::visualization::PCLVisualizer::Ptr viewer;
if (pcl::console::find_argument (argc, argv, "-f") >= 0 || pcl::console::find_argument (argc, argv, "-sf") >= 0)
viewer = simpleVis(final);
else
viewer = simpleVis(cloud);
while (!viewer->wasStopped ())
{
viewer->spinOnce (100);
std::this_thread::sleep_for(100ms);
}
return 0;
}
2.2 CMakeLists.txt
cmake_minimum_required(VERSION 3.5 FATAL_ERROR)
project(random_sample_consensus)
find_package(PCL 1.2 REQUIRED)
include_directories(${PCL_INCLUDE_DIRS})
link_directories(${PCL_LIBRARY_DIRS})
add_definitions(${PCL_DEFINITIONS})
add_executable (${PROJECT_NAME} RandomSampleConsensusModel.cpp)
target_link_libraries (${PROJECT_NAME} ${PCL_LIBRARIES})
3、代码运行结果
- 编译
mkdir build && cd build
cmake ..
make
3.1 原始点云
- 运行1(不做处理),显示原始点云,可以看出点云的组织很少,而且它包含许多异常值。
./random_sample_consensus
3.2 平面模型
- 运行2,选择的特定模型(在本例中是
平面
),可以看到所有点云都在一个平面模型内。
./random_sample_consensus -f
3.3 球形模型
- 运行3,选择的特定模型(在本例中是
球形
),可以看到所有点云都在一个平面模型内。
./random_sample_consensus -sf
4、代码解读
代码通过 RANSAC(随机样本一致性)算法,用于从点云数据中拟合平面或球体模型。以下是代码的详细解读:
4.1 可视化函数 simpleVis
pcl::visualization::PCLVisualizer::Ptr
simpleVis (pcl::PointCloud<pcl::PointXYZ>::ConstPtr cloud)
{
pcl::visualization::PCLVisualizer::Ptr viewer (new pcl::visualization::PCLVisualizer ("3D Viewer"));
viewer->setBackgroundColor (0, 0, 0);
viewer->addPointCloud<pcl::PointXYZ> (cloud, "sample cloud");
viewer->setPointCloudRenderingProperties (pcl::visualization::PCL_VISUALIZER_POINT_SIZE, 3, "sample cloud");
viewer->initCameraParameters ();
return (viewer);
}
- 功能:创建一个 3D 可视化窗口,并将点云数据添加到窗口中。
- 参数:
cloud
:输入的点云数据。
- 实现细节:
- 设置背景颜色为黑色。
- 添加点云并设置点的大小为 3。
- 初始化相机参数。
4.2 初始化点云
pcl::PointCloud<pcl::PointXYZ>::Ptr cloud (new pcl::PointCloud<pcl::PointXYZ>);
pcl::PointCloud<pcl::PointXYZ>::Ptr final (new pcl::PointCloud<pcl::PointXYZ>);
cloud->width = 500;
cloud->height = 1;
cloud->is_dense = false;
cloud->points.resize (cloud->width * cloud->height);
- 创建一个包含 500 个点的点云对象
cloud
,并初始化其属性。 final
用于存储 RANSAC 拟合后的内点。
4.3 生成随机点云数据
for (pcl::index_t i = 0; i < static_cast<pcl::index_t>(cloud->size ()); ++i)
{
if (pcl::console::find_argument (argc, argv, "-s") >= 0 || pcl::console::find_argument (argc, argv, "-sf") >= 0)
{
(*cloud)[i].x = 1024 * rand () / (RAND_MAX + 1.0);
(*cloud)[i].y = 1024 * rand () / (RAND_MAX + 1.0);
if (i % 5 == 0)
(*cloud)[i].z = 1024 * rand () / (RAND_MAX + 1.0);
else if(i % 2 == 0)
(*cloud)[i].z = sqrt( 1 - ((*cloud)[i].x * (*cloud)[i].x)
- ((*cloud)[i].y * (*cloud)[i].y));
else
(*cloud)[i].z = - sqrt( 1 - ((*cloud)[i].x * (*cloud)[i].x)
- ((*cloud)[i].y * (*cloud)[i].y));
}
else
{
(*cloud)[i].x = 1024 * rand () / (RAND_MAX + 1.0);
(*cloud)[i].y = 1024 * rand () / (RAND_MAX + 1.0);
if( i % 2 == 0)
(*cloud)[i].z = 1024 * rand () / (RAND_MAX + 1.0);
else
(*cloud)[i].z = -1 * ((*cloud)[i].x + (*cloud)[i].y);
}
}
- 功能:生成随机点云数据。
- 逻辑:
- 如果命令行参数包含
-s
或-sf
,生成球体模型的点云数据。 - 否则,生成平面模型的点云数据。
- 如果命令行参数包含
- 球体模型:
- 大部分点分布在球体表面(通过
sqrt(1 - x² - y²)
计算)。 - 少量点随机分布在空间中(
i % 5 == 0
时随机生成)。
- 大部分点分布在球体表面(通过
- 平面模型:
- 点的
z
值由- (x + y)
决定,形成一个平面。
- 点的
4.4 RANSAC 模型拟合
std::vector<int> inliers;
pcl::SampleConsensusModelSphere<pcl::PointXYZ>::Ptr
model_s(new pcl::SampleConsensusModelSphere<pcl::PointXYZ> (cloud));
pcl::SampleConsensusModelPlane<pcl::PointXYZ>::Ptr
model_p (new pcl::SampleConsensusModelPlane<pcl::PointXYZ> (cloud));
if(pcl::console::find_argument (argc, argv, "-f") >= 0)
{
pcl::RandomSampleConsensus<pcl::PointXYZ> ransac (model_p);
ransac.setDistanceThreshold (.01);
ransac.computeModel();
ransac.getInliers(inliers);
}
else if (pcl::console::find_argument (argc, argv, "-sf") >= 0 )
{
pcl::RandomSampleConsensus<pcl::PointXYZ> ransac (model_s);
ransac.setDistanceThreshold (.01);
ransac.computeModel();
ransac.getInliers(inliers);
}
- 功能:使用 RANSAC 拟合平面或球体模型。
- 逻辑:
- 如果命令行参数包含
-f
,拟合平面模型。 - 如果命令行参数包含
-sf
,拟合球体模型。
- 如果命令行参数包含
- 关键参数:
setDistanceThreshold(.01)
:设置内点的距离阈值。computeModel()
:计算模型。getInliers(inliers)
:获取内点的索引。
4.5 提取内点
pcl::copyPointCloud (*cloud, inliers, *final);
- 将 RANSAC 找到的内点从原始点云复制到
final
点云中。
4.6 可视化
pcl::visualization::PCLVisualizer::Ptr viewer;
if (pcl::console::find_argument (argc, argv, "-f") >= 0 || pcl::console::find_argument (argc, argv, "-sf") >= 0)
viewer = simpleVis(final);
else
viewer = simpleVis(cloud);
while (!viewer->wasStopped ())
{
viewer->spinOnce (100);
std::this_thread::sleep_for(100ms);
}
- 功能:可视化点云。
- 逻辑:
- 如果命令行参数包含
-f
或-sf
,显示拟合后的内点。 - 否则,显示原始点云。
- 如果命令行参数包含
- 刷新窗口:每 100 毫秒刷新一次。