Python 网络爬虫入门与实践:从基础到高级技巧
文章目录
- 1. 简介
- 2. 环境配置
- 3. 网络爬虫基础知识
- 什么是网络爬虫?
- 网络爬虫的类型
- 网络爬虫的工作原理
- 网络爬虫的合法性
- 4. 常用 Python 库介绍
- Requests
- BeautifulSoup
- lxml
- Scrapy
- 5. 实战案例
- 案例 1:爬取豆瓣电影 Top250
- 案例 2:爬取青春有你 2 选手照片
- 6. 高级技巧
- 并发抓取
- 动态内容处理
- 反爬虫策略
- 7. 注意事项与最佳实践
- 8. 总结
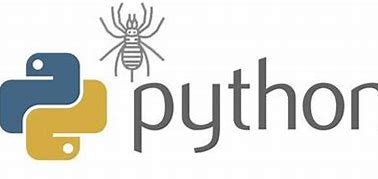
1. 简介
网络爬虫(Web Crawler)是一种自动化程序,用于从互联网上抓取数据。它通过模拟浏览器请求,访问网页并提取所需的信息。网络爬虫广泛应用于搜索引擎、数据挖掘、市场分析等领域。
在本博客中,我们将从基础概念入手,逐步深入,探讨如何使用 Python 编写网络爬虫。我们将介绍常用的 Python 库,并通过实战案例演示如何爬取网页数据。最后,我们还将讨论一些高级技巧和注意事项,帮助你编写高效、合法的网络爬虫。
2. 环境配置
在开始编写网络爬虫之前,我们需要配置 Python 环境并安装必要的库。以下是推荐的开发环境:
- Python 3.x:建议使用 Python 3.7 或更高版本。
- IDE:推荐使用 PyCharm、VS Code 或 Jupyter Notebook。
- 库安装:使用
pip
安装以下库:
pip install requests beautifulsoup4 lxml scrapy
3. 网络爬虫基础知识
什么是网络爬虫?
网络爬虫是一种自动化程序,用于从互联网上抓取数据。它通过模拟浏览器请求,访问网页并提取所需的信息。网络爬虫广泛应用于搜索引擎、数据挖掘、市场分析等领域。
网络爬虫的类型
- 通用爬虫:用于抓取整个互联网的数据,如搜索引擎的爬虫。
- 聚焦爬虫:针对特定网站或主题进行抓取,如电商价格监控。
- 增量式爬虫:只抓取更新的内容,减少重复抓取的开销。
- 深层爬虫:抓取隐藏在深层网页中的数据,如表单提交后的结果。
网络爬虫的工作原理
- 发送请求:爬虫向目标网站发送 HTTP 请求,获取网页内容。
- 解析内容:使用 HTML 解析器提取所需的数据。
- 存储数据:将提取的数据保存到文件或数据库中。
- 处理链接:从当前页面提取其他链接,继续抓取。
网络爬虫的合法性
网络爬虫的合法性取决于其用途和抓取方式。以下是一些需要注意的法律和道德问题:
- 遵守
robots.txt
:该文件定义了网站允许或禁止爬虫访问的页面。 - 设置延迟:避免频繁请求,给服务器带来负担。
- 尊重版权:不要抓取受版权保护的内容。
- 隐私保护:不要抓取用户的个人信息。
4. 常用 Python 库介绍
Requests
Requests
是一个简单易用的 HTTP 库,用于发送 HTTP 请求。它支持 GET、POST 等多种请求方法,并可以设置请求头、参数等。
import requests
response = requests.get('https://www.example.com')
print(response.text)
BeautifulSoup
BeautifulSoup
是一个 HTML 解析库,用于从网页中提取数据。它支持多种解析器,如 lxml
、html.parser
等。
from bs4 import BeautifulSoup
html_doc = """
<html><head><title>Example</title></head>
<body><p>Hello, World!</p></body></html>
"""
soup = BeautifulSoup(html_doc, 'lxml')
print(soup.title.text) # 输出: Example
lxml
lxml
是一个高性能的 XML 和 HTML 解析库,支持 XPath 和 CSS 选择器。
from lxml import etree
html_doc = """
<html><head><title>Example</title></head>
<body><p>Hello, World!</p></body></html>
"""
tree = etree.HTML(html_doc)
title = tree.xpath('//title/text()')
print(title) # 输出: ['Example']
Scrapy
Scrapy
是一个强大的爬虫框架,支持并发抓取、数据存储、中间件等功能。它适合大规模的数据抓取任务。
pip install scrapy
import scrapy
class ExampleSpider(scrapy.Spider):
name = 'example'
start_urls = ['https://www.example.com']
def parse(self, response):
title = response.css('title::text').get()
yield {'title': title}
5. 实战案例
案例 1:爬取豆瓣电影 Top250
在这个案例中,我们将使用 Requests
和 BeautifulSoup
爬取豆瓣电影 Top250 的电影名称和评分。
import requests
from bs4 import BeautifulSoup
url = 'https://movie.douban.com/top250'
headers = {
'User-Agent': 'Mozilla/5.0 (Windows NT 10.0; Win64; x64) AppleWebKit/537.36 (KHTML, like Gecko) Chrome/58.0.3029.110 Safari/537.3'
}
response = requests.get(url, headers=headers)
soup = BeautifulSoup(response.text, 'lxml')
movies = soup.find_all('div', class_='hd')
for movie in movies:
title = movie.a.span.text
print(title)
案例 2:爬取青春有你 2 选手照片
在这个案例中,我们将爬取青春有你 2 选手的照片,并保存到本地。
import requests
from bs4 import BeautifulSoup
import os
url = 'https://www.example.com/qingchunniyou2'
headers = {
'User-Agent': 'Mozilla/5.0 (Windows NT 10.0; Win64; x64) AppleWebKit/537.36 (KHTML, like Gecko) Chrome/58.0.3029.110 Safari/537.3'
}
response = requests.get(url, headers=headers)
soup = BeautifulSoup(response.text, 'lxml')
images = soup.find_all('img', class_='contestant-image')
if not os.path.exists('images'):
os.makedirs('images')
for img in images:
img_url = img['src']
img_data = requests.get(img_url).content
with open(f'images/{img_url.split("/")[-1]}', 'wb') as f:
f.write(img_data)
6. 高级技巧
并发抓取
使用 concurrent.futures
或 Scrapy
实现并发抓取,提高爬虫效率。
from concurrent.futures import ThreadPoolExecutor
import requests
urls = ['https://www.example.com/page1', 'https://www.example.com/page2']
def fetch(url):
response = requests.get(url)
return response.text
with ThreadPoolExecutor(max_workers=5) as executor:
results = executor.map(fetch, urls)
for result in results:
print(result)
动态内容处理
使用 Selenium
或 Playwright
处理动态加载的内容。
from selenium import webdriver
driver = webdriver.Chrome()
driver.get('https://www.example.com')
content = driver.page_source
print(content)
driver.quit()
反爬虫策略
- 设置请求头:模拟浏览器请求。
- 使用代理:避免 IP 被封禁。
- 设置延迟:降低请求频率。
- 处理验证码:使用 OCR 或手动输入。
**
**
7. 注意事项与最佳实践
- 遵守
robots.txt
:尊重网站的爬虫政策。 - 设置合理的延迟:避免给服务器带来负担。
- 处理异常:增加异常处理机制,确保爬虫的稳定性。
- 数据存储:选择合适的数据存储方式,如文件、数据库等。
- 日志记录:记录爬虫的运行状态,便于调试和监控。
8. 总结
我们详细介绍了 Python 网络爬虫的基础知识、常用库、实战案例以及高级技巧。希望这些内容能帮助你快速入门并掌握网络爬虫的开发技能。在实际应用中,请务必遵守相关法律法规,尊重网站的爬虫政策,编写合法、高效的网络爬虫。
参考内容:
- Requests 官方文档
- BeautifulSoup 官方文档
- Scrapy 官方文档
- Selenium 官方文档