【开放词汇检测】基于MMDetection的MM-Grounding-DINO实战
文章目录
- 摘要
- 安装基础环境
- 新建虚拟环境
- 安装pytorch
- 安装openmim、mmengine、mmcv
- 安装 MMDetection
- 验证安装
- 配置OV-DINO环境
- MMDetection的MM-Grounding-DINO详细介绍
- 测试结果
- Zero-Shot COCO 结果与模型
- Zero-Shot LVIS Results
- Zero-Shot ODinW(野生环境下的目标检测)结果
- ODinW13的结果和模型
- ODinW35的结果和模型
- 零样本指代表达式理解结果
- 零样本描述检测数据集(DOD)
- Pretrain Flickr30k Results
- 通过微调验证预训练模型的泛化能力
- RTTS
- RUOD
- Brain Tumor
- Cityscapes
- People in Painting
- COCO
- LVIS 1.0
- RefEXP
- RefCOCO
- RefCOCO+
- RefCOCOg
- gRefCOCO
- MM-GDINO-T 预训练数据准备和处理
- 用到的数据集
- 1 Objects365 v1
- 2 COCO 2017
- 3 GoldG
- 4 GRIT-20M
- 5 V3Det
- 6 数据切分和可视化
- MM-GDINO-L 预训练数据准备和处理
- 用到的数据集
- 1 Object365 v2
- 2 OpenImages v6
- 3 V3Det
- 4 LVIS 1.0
- 5 COCO2017 OD
- 6 GoldG
- 7 COCO2014 VG
- 8 Referring Expression Comprehension
- 9 GRIT-20M
- 评测数据集准备
- 1 COCO 2017
- 2 LVIS 1.0
- 3 ODinW
- 4 DOD
- 5 Flickr30k Entities
- 6 Referring Expression Comprehension
- 微调数据集准备
- 1 COCO 2017
- 2 LVIS 1.0
- 3 RTTS
- 4 RUOD
- 5 Brain Tumor
- 6 Cityscapes
- 7 People in Painting
- 8 Referring Expression Comprehension
- 推理与微调
- MM Grounding DINO-T 模型权重下载
- 推理
- 评测
- 评测数据集结果可视化
- 模型训练
- 预训练自定义格式说明
- 自定义数据集微调训练案例
- 1 数据准备
- 2 配置准备
- 3 可视化和 Zero-Shot 评估
- 4 模型训练
- 模型自训练伪标签迭代生成和优化 pipeline
- 1 目标检测格式
- 2 Phrase Grounding 格式
摘要
基础环境:Ubuntu 22.04、CUDA 11.7
安装基础环境
新建虚拟环境
conda create --name openmm python=3.9
输入y。
安装pytorch
conda install pytorch==2.0.0 torchvision==0.15.0 torchaudio==2.0.0 pytorch-cuda=11.7 -c pytorch -c nvidia
安装openmim、mmengine、mmcv
pip install -U openmim
mim install mmengine
mim install "mmcv==2.0.0rc4"
这里不要用>=,如果使用了默认安装最新版本,不兼容!所以,使用==安装最低要求的版本即可!!
注意: 在 MMCV-v2.x 中,mmcv-full
改名为 mmcv
,如果你想安装不包含 CUDA 算子精简版,可以通过 mim install "mmcv-lite>=2.0.0rc1"
来安装。
安装编译mmcv时间很长,如上图,如果不想安装编译,可以使用编译好的库,链接:
https://mmcv.readthedocs.io/en/latest/get_started/installation.html
安装本机的环境安装编译!
安装 MMDetection
下载MMdetection,代码链接:https://github.com/open-mmlab/mmdetection
, 下载后解压进入到根目录。或者直接使用git获取源码,如下:
git clone https://github.com/open-mmlab/mmdetection.git
cd mmdetection
pip install -v -e .
# "-v" 指详细说明,或更多的输出
# "-e" 表示在可编辑模式下安装项目,因此对代码所做的任何本地修改都会生效,从而无需重新安装。
或者将 mmdet 作为依赖或第三方 Python 包,使用 MIM 安装:
mim install mmdet
验证安装
为了验证 MMDetection 是否安装正确,我们提供了一些示例代码来执行模型推理。
步骤 1. 我们需要下载配置文件和模型权重文件。
mim download mmdet --config rtmdet_tiny_8xb32-300e_coco --dest .
下载将需要几秒钟或更长时间,这取决于你的网络环境。完成后,你会在当前文件夹中发现两个文件 rtmdet_tiny_8xb32-300e_coco.py
和 rtmdet_tiny_8xb32-300e_coco_20220902_112414-78e30dcc.pth
。
步骤 2. 推理验证。
如果你通过源码安装的 MMDetection,那么直接运行以下命令进行验证:
python demo/image_demo.py demo/demo.jpg rtmdet_tiny_8xb32-300e_coco.py --weights rtmdet_tiny_8xb32-300e_coco_20220902_112414-78e30dcc.pth --device cpu
你会在当前文件夹中的 outputs/vis
文件夹中看到一个新的图像 demo.jpg
,图像中包含有网络预测的检测框。
如果你通过 MIM 安装的 MMDetection,那么可以打开你的 Python 解析器,复制并粘贴以下代码:
from mmdet.apis import init_detector, inference_detector
import mmcv
# 指定模型的配置文件和 checkpoint 文件路径
config_file = 'configs/faster_rcnn/faster_rcnn_r50_fpn_1x_coco.py'
checkpoint_file = 'checkpoints/faster_rcnn_r50_fpn_1x_coco_20200130-047c8118.pth'
# 根据配置文件和 checkpoint 文件构建模型
model = init_detector(config_file, checkpoint_file, device='cuda:0')
# 测试单张图片并展示结果
img = 'test.jpg' # 或者 img = mmcv.imread(img),这样图片仅会被读一次
result = inference_detector(model, img)
# 在一个新的窗口中将结果可视化
model.show_result(img, result)
# 或者将可视化结果保存为图片
model.show_result(img, result, out_file='result.jpg')
# 测试视频并展示结果
video = mmcv.VideoReader('video.mp4')
for frame in video:
result = inference_detector(model, frame)
model.show_result(frame, result, wait_time=1)
你将会看到一个包含 DetDataSample
的列表,预测结果在 pred_instance
里,包含有检测框,类别和得分。
配置OV-DINO环境
安装OV-DINO需要用到的库文件,如下:
首先安装numpy库,默认安装是2.x的版本,不能用,需要切换到1.x的版本。命令如下:
pip install numpy==1.24.3
安装其他的库文件,命令如下:
pip install terminaltables
pip install pycocotools
pip install shapely
pip install scipy
pip install fairscale
安装Transformer,由于用到了Bert,所以要安装Transformer,安装命令
pip install transformers
由于国内不能直接链接huggingface,所以需要用到代理:设置方式如下:
在image_demo脚本中加入代理链接,代码如下:
import os
os.environ["HF_ENDPOINT"] = "https://hf-mirror.com"
然后,运行命令:
python demo/image_demo.py demo/0016.jpg configs/mm_grounding_dino/grounding_dino_swin-b_pretrain_obj365_goldg_v3det.py --weights grounding_dino_swin-b_pretrain_all-f9818a7c.pth --texts 'the standing man . the squatting man' -c --device 'cuda:1'
MMDetection的MM-Grounding-DINO详细介绍
Grounding-DINO 是一种先进的开放集检测模型,能够处理包括开放词汇检测(OVD)、短语定位(PG)和指代表达式理解(REC)在内的多种视觉任务。由于其有效性,Grounding-DINO 已被广泛采用为各种下游应用的主流架构。然而,尽管它意义重大,但由于训练代码的不可用性,原始的 Grounding-DINO 模型缺乏全面的公共技术细节。为了弥补这一差距,我们推出了 MM-Grounding-DINO,这是一个基于 MMDetection 工具箱构建的开源、全面且用户友好的基线。它采用了丰富的视觉数据集进行预训练,并利用各种检测和定位数据集进行微调。我们对每个报告的结果进行了全面分析,并提供了详细的设置以便复现。在提到的基准测试上的广泛实验表明,我们的 MM-Grounding-DINO-Tiny 优于 Grounding-DINO-Tiny 基线。我们已将所有模型向研究界公开。
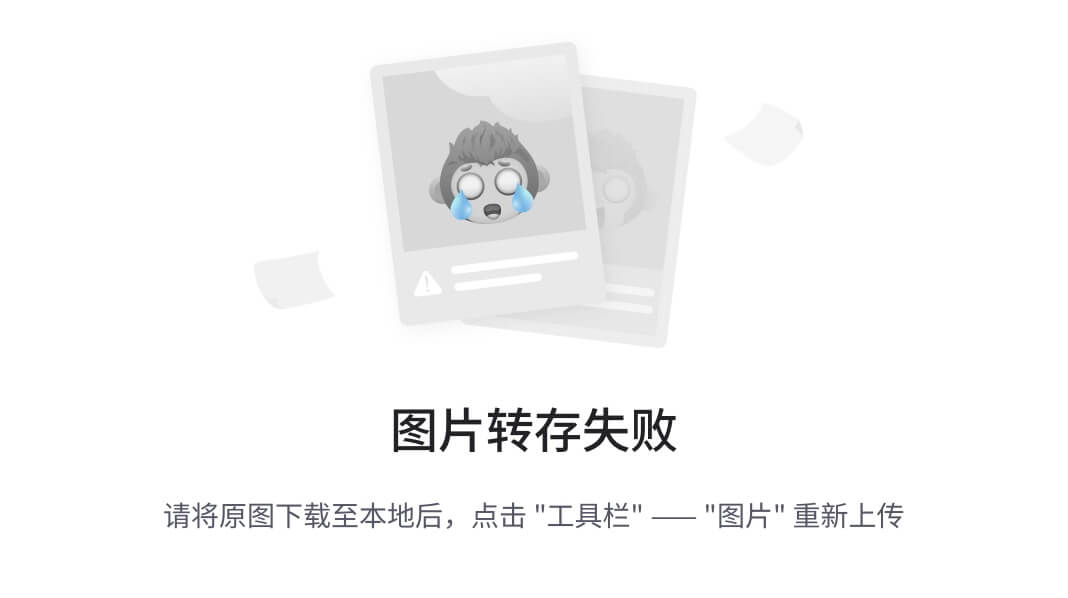
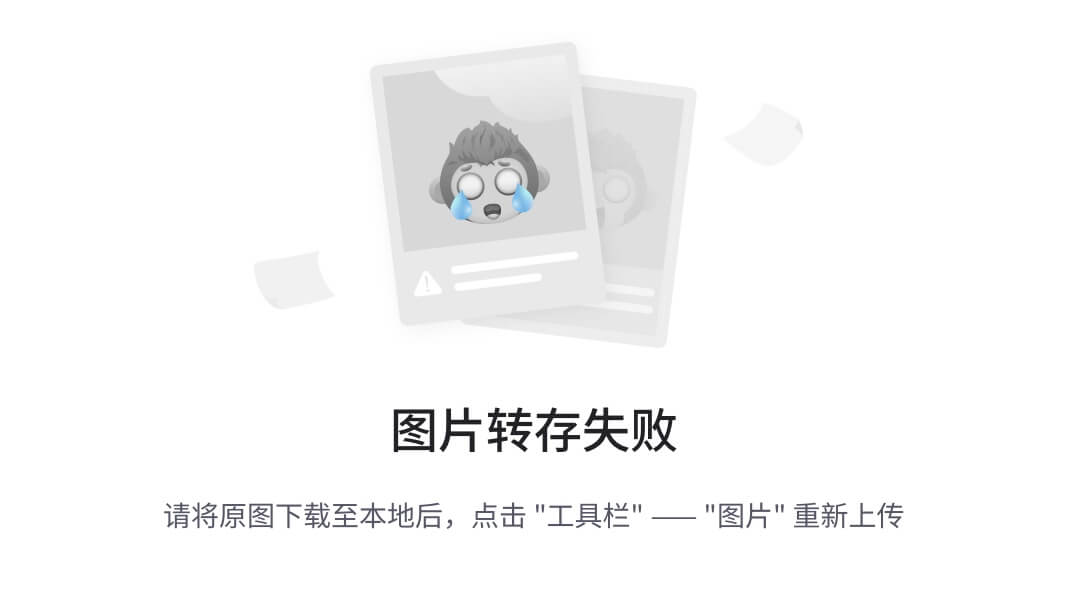
测试结果
Zero-Shot COCO 结果与模型
Model | Backbone | Style | COCO mAP | Pre-Train Data | Config | Download |
---|---|---|---|---|---|---|
GDINO-T | Swin-T | Zero-shot | 46.7 | O365 | ||
GDINO-T | Swin-T | Zero-shot | 48.1 | O365,GoldG | ||
GDINO-T | Swin-T | Zero-shot | 48.4 | O365,GoldG,Cap4M | config | model |
MM-GDINO-T | Swin-T | Zero-shot | 48.5(+1.8) | O365 | config | |
MM-GDINO-T | Swin-T | Zero-shot | 50.4(+2.3) | O365,GoldG | config | model | log |
MM-GDINO-T | Swin-T | Zero-shot | 50.5(+2.1) | O365,GoldG,GRIT | config | model | log |
MM-GDINO-T | Swin-T | Zero-shot | 50.6(+2.2) | O365,GoldG,V3Det | config | model | log |
MM-GDINO-T | Swin-T | Zero-shot | 50.4(+2.0) | O365,GoldG,GRIT,V3Det | config | model | log |
MM-GDINO-B | Swin-B | Zero-shot | 52.5 | O365,GoldG,V3Det | config | model | log |
MM-GDINO-B* | Swin-B | - | 59.5 | O365,ALL | config | model | log |
MM-GDINO-L | Swin-L | Zero-shot | 53.0 | O365V2,OpenImageV6,GoldG | config | model | log |
MM-GDINO-L* | Swin-L | - | 60.3 | O365V2,OpenImageV6,ALL | config | model | log |
- 这个*表示模型尚未完全训练。我们将在未来发布最终权重。
- ALL: GoldG,V3det,COCO2017,LVISV1,COCO2014,GRIT,RefCOCO,RefCOCO+,RefCOCOg,gRefCOCO。
Zero-Shot LVIS Results
Model | MiniVal APr | MiniVal APc | MiniVal APf | MiniVal AP | Val1.0 APr | Val1.0 APc | Val1.0 APf | Val1.0 AP | Pre-Train Data |
---|---|---|---|---|---|---|---|---|---|
GDINO-T | 18.8 | 24.2 | 34.7 | 28.8 | 10.1 | 15.3 | 29.9 | 20.1 | O365,GoldG,Cap4M |
MM-GDINO-T | 28.1 | 30.2 | 42.0 | 35.7(+6.9) | 17.1 | 22.4 | 36.5 | 27.0(+6.9) | O365,GoldG |
MM-GDINO-T | 26.6 | 32.4 | 41.8 | 36.5(+7.7) | 17.3 | 22.6 | 36.4 | 27.1(+7.0) | O365,GoldG,GRIT |
MM-GDINO-T | 33.0 | 36.0 | 45.9 | 40.5(+11.7) | 21.5 | 25.5 | 40.2 | 30.6(+10.5) | O365,GoldG,V3Det |
MM-GDINO-T | 34.2 | 37.4 | 46.2 | 41.4(+12.6) | 23.6 | 27.6 | 40.5 | 31.9(+11.8) | O365,GoldG,GRIT,V3Det |
- MM-GDINO-T的配置文件是mini-lvis和lvis 1.0
Zero-Shot ODinW(野生环境下的目标检测)结果
ODinW13的结果和模型
Method | GDINO-T (O365,GoldG,Cap4M) | MM-GDINO-T (O365,GoldG) | MM-GDINO-T (O365,GoldG,GRIT) | MM-GDINO-T (O365,GoldG,V3Det) | MM-GDINO-T (O365,GoldG,GRIT,V3Det) |
---|---|---|---|---|---|
AerialMaritimeDrone | 0.173 | 0.133 | 0.155 | 0.177 | 0.151 |
Aquarium | 0.195 | 0.252 | 0.261 | 0.266 | 0.283 |
CottontailRabbits | 0.799 | 0.771 | 0.810 | 0.778 | 0.786 |
EgoHands | 0.608 | 0.499 | 0.537 | 0.506 | 0.519 |
NorthAmericaMushrooms | 0.507 | 0.331 | 0.462 | 0.669 | 0.767 |
Packages | 0.687 | 0.707 | 0.687 | 0.710 | 0.706 |
PascalVOC | 0.563 | 0.565 | 0.580 | 0.556 | 0.566 |
pistols | 0.726 | 0.585 | 0.709 | 0.671 | 0.729 |
pothole | 0.215 | 0.136 | 0.285 | 0.199 | 0.243 |
Raccoon | 0.549 | 0.469 | 0.511 | 0.553 | 0.535 |
ShellfishOpenImages | 0.393 | 0.321 | 0.437 | 0.519 | 0.488 |
thermalDogsAndPeople | 0.657 | 0.556 | 0.603 | 0.493 | 0.542 |
VehiclesOpenImages | 0.613 | 0.566 | 0.603 | 0.614 | 0.615 |
Average | 0.514 | 0.453 | 0.511 | 0.516 | 0.533 |
- MM-GDINO-T的配置文件是odinw13
ODinW35的结果和模型
Method | GDINO-T (O365,GoldG,Cap4M) | MM-GDINO-T (O365,GoldG) | MM-GDINO-T (O365,GoldG,GRIT) | MM-GDINO-T (O365,GoldG,V3Det) | MM-GDINO-T (O365,GoldG,GRIT,V3Det) |
---|---|---|---|---|---|
AerialMaritimeDrone_large | 0.173 | 0.133 | 0.155 | 0.177 | 0.151 |
AerialMaritimeDrone_tiled | 0.206 | 0.170 | 0.225 | 0.184 | 0.206 |
AmericanSignLanguageLetters | 0.002 | 0.016 | 0.020 | 0.011 | 0.007 |
Aquarium | 0.195 | 0.252 | 0.261 | 0.266 | 0.283 |
BCCD | 0.161 | 0.069 | 0.118 | 0.083 | 0.077 |
boggleBoards | 0.000 | 0.002 | 0.001 | 0.001 | 0.002 |
brackishUnderwater | 0.021 | 0.033 | 0.021 | 0.025 | 0.025 |
ChessPieces | 0.000 | 0.000 | 0.000 | 0.000 | 0.000 |
CottontailRabbits | 0.806 | 0.771 | 0.810 | 0.778 | 0.786 |
dice | 0.004 | 0.002 | 0.005 | 0.001 | 0.001 |
DroneControl | 0.042 | 0.047 | 0.097 | 0.088 | 0.074 |
EgoHands_generic | 0.608 | 0.527 | 0.537 | 0.506 | 0.519 |
EgoHands_specific | 0.002 | 0.001 | 0.005 | 0.007 | 0.003 |
HardHatWorkers | 0.046 | 0.048 | 0.070 | 0.070 | 0.108 |
MaskWearing | 0.004 | 0.009 | 0.004 | 0.011 | 0.009 |
MountainDewCommercial | 0.430 | 0.453 | 0.465 | 0.194 | 0.430 |
NorthAmericaMushrooms | 0.471 | 0.331 | 0.462 | 0.669 | 0.767 |
openPoetryVision | 0.000 | 0.001 | 0.000 | 0.000 | 0.000 |
OxfordPets_by_breed | 0.003 | 0.002 | 0.004 | 0.006 | 0.004 |
OxfordPets_by_species | 0.011 | 0.019 | 0.016 | 0.020 | 0.015 |
PKLot | 0.001 | 0.004 | 0.002 | 0.008 | 0.007 |
Packages | 0.695 | 0.707 | 0.687 | 0.710 | 0.706 |
PascalVOC | 0.563 | 0.565 | 0.580 | 0.566 | 0.566 |
pistols | 0.726 | 0.585 | 0.709 | 0.671 | 0.729 |
plantdoc | 0.005 | 0.005 | 0.007 | 0.008 | 0.011 |
pothole | 0.215 | 0.136 | 0.219 | 0.077 | 0.168 |
Raccoons | 0.549 | 0.469 | 0.511 | 0.553 | 0.535 |
selfdrivingCar | 0.089 | 0.091 | 0.076 | 0.094 | 0.083 |
ShellfishOpenImages | 0.393 | 0.321 | 0.437 | 0.519 | 0.488 |
ThermalCheetah | 0.087 | 0.063 | 0.081 | 0.030 | 0.045 |
thermalDogsAndPeople | 0.657 | 0.556 | 0.603 | 0.493 | 0.543 |
UnoCards | 0.006 | 0.012 | 0.010 | 0.009 | 0.005 |
VehiclesOpenImages | 0.613 | 0.566 | 0.603 | 0.614 | 0.615 |
WildfireSmoke | 0.134 | 0.106 | 0.154 | 0.042 | 0.127 |
websiteScreenshots | 0.012 | 0.02 | 0.016 | 0.016 | 0.016 |
Average | 0.227 | 0.202 | 0.228 | 0.214 | 0.284 |
- MM-GDINO-T的配置文件是odinw35
零样本指代表达式理解结果
Method | GDINO-T (O365,GoldG,Cap4M) | MM-GDINO-T (O365,GoldG) | MM-GDINO-T (O365,GoldG,GRIT) | MM-GDINO-T (O365,GoldG,V3Det) | MM-GDINO-T (O365,GoldG,GRIT,V3Det) |
---|---|---|---|---|---|
RefCOCO val @1,5,10 | 50.8/89.5/94.9 | 53.1/89.9/94.7 | 53.4/90.3/95.5 | 52.1/89.8/95.0 | 53.1/89.7/95.1 |
RefCOCO testA @1,5,10 | 57.4/91.3/95.6 | 59.7/91.5/95.9 | 58.8/91.70/96.2 | 58.4/86.8/95.6 | 59.1/91.0/95.5 |
RefCOCO testB @1,5,10 | 45.0/86.5/92.9 | 46.4/86.9/92.2 | 46.8/87.7/93.3 | 45.4/86.2/92.6 | 46.8/87.8/93.6 |
RefCOCO+ val @1,5,10 | 51.6/86.4/92.6 | 53.1/87.0/92.8 | 53.5/88.0/93.7 | 52.5/86.8/93.2 | 52.7/87.7/93.5 |
RefCOCO+ testA @1,5,10 | 57.3/86.7/92.7 | 58.9/87.3/92.9 | 59.0/88.1/93.7 | 58.1/86.7/93.5 | 58.7/87.2/93.1 |
RefCOCO+ testB @1,5,10 | 46.4/84.1/90.7 | 47.9/84.3/91.0 | 47.9/85.5/92.7 | 46.9/83.7/91.5 | 48.4/85.8/92.1 |
RefCOCOg val @1,5,10 | 60.4/92.1/96.2 | 61.2/92.6/96.1 | 62.7/93.3/97.0 | 61.7/92.9/96.6 | 62.9/93.3/97.2 |
RefCOCOg test @1,5,10 | 59.7/92.1/96.3 | 61.1/93.3/96.7 | 62.6/94.9/97.1 | 61.0/93.1/96.8 | 62.9/93.9/97.4 |
Method | thresh_score | GDINO-T (O365,GoldG,Cap4M) | MM-GDINO-T (O365,GoldG) | MM-GDINO-T (O365,GoldG,GRIT) | MM-GDINO-T (O365,GoldG,V3Det) | MM-GDINO-T (O365,GoldG,GRIT,V3Det) |
---|---|---|---|---|---|---|
gRefCOCO val Pr@(F1=1, IoU≥0.5),N-acc | 0.5 | 39.3/70.4 | 39.4/67.5 | |||
gRefCOCO val Pr@(F1=1, IoU≥0.5),N-acc | 0.6 | 40.5/83.8 | 40.6/83.1 | |||
gRefCOCO val Pr@(F1=1, IoU≥0.5),N-acc | 0.7 | 41.3/91.8 | 39.8/84.7 | 40.7/89.7 | 40.3/88.8 | 41.0/91.3 |
gRefCOCO val Pr@(F1=1, IoU≥0.5),N-acc | 0.8 | 41.5/96.8 | 41.1/96.4 | |||
gRefCOCO testA Pr@(F1=1, IoU≥0.5),N-acc | 0.5 | 31.9/70.4 | 33.1/69.5 | |||
gRefCOCO testA Pr@(F1=1, IoU≥0.5),N-acc | 0.6 | 29.3/82.9 | 29.2/84.3 | |||
gRefCOCO testA Pr@(F1=1, IoU≥0.5),N-acc | 0.7 | 27.2/90.2 | 26.3/89.0 | 26.0/91.9 | 25.4/91.8 | 26.1/93.0 |
gRefCOCO testA Pr@(F1=1, IoU≥0.5),N-acc | 0.8 | 25.1/96.3 | 23.8/97.2 | |||
gRefCOCO testB Pr@(F1=1, IoU≥0.5),N-acc | 0.5 | 30.9/72.5 | 33.0/69.6 | |||
gRefCOCO testB Pr@(F1=1, IoU≥0.5),N-acc | 0.6 | 30.0/86.1 | 31.6/96.7 | |||
gRefCOCO testB Pr@(F1=1, IoU≥0.5),N-acc | 0.7 | 29.7/93.5 | 31.3/84.8 | 30.6/90.2 | 30.7/89.9 | 30.4/92.3 |
gRefCOCO testB Pr@(F1=1, IoU≥0.5),N-acc | 0.8 | 29.1/97.4 | 29.5/84.2 |
- MM-GDINO-T的配置文件位于:refcoco/grounding_dino_swin-t_pretrain_zeroshot_refexp.py
零样本描述检测数据集(DOD)
pip install ddd-dataset
Method | mode | GDINO-T (O365,GoldG,Cap4M) | MM-GDINO-T (O365,GoldG) | MM-GDINO-T (O365,GoldG,GRIT) | MM-GDINO-T (O365,GoldG,V3Det) | MM-GDINO-T (O365,GoldG,GRIT,V3Det) |
---|---|---|---|---|---|---|
FULL/short/middle/long/very long | concat | 17.2/18.0/18.7/14.8/16.3 | 15.6/17.3/16.7/14.3/13.1 | 17.0/17.7/18.0/15.7/15.7 | 16.2/17.4/16.8/14.9/15.4 | 17.5/23.4/18.3/14.7/13.8 |
FULL/short/middle/long/very long | parallel | 22.3/28.2/24.8/19.1/13.9 | 21.7/24.7/24.0/20.2/13.7 | 22.5/25.6/25.1/20.5/14.9 | 22.3/25.6/24.5/20.6/14.7 | 22.9/28.1/25.4/20.4/14.4 |
PRES/short/middle/long/very long | concat | 17.8/18.3/19.2/15.2/17.3 | 16.4/18.4/17.3/14.5/14.2 | 17.9/19.0/18.3/16.5/17.5 | 16.6/18.8/17.1/15.1/15.0 | 18.0/23.7/18.6/15.4/13.3 |
PRES/short/middle/long/very long | parallel | 21.0/27.0/22.8/17.5/12.5 | 21.3/25.5/22.8/19.2/12.9 | 21.5/25.2/23.0/19.0/15.0 | 21.6/25.7/23.0/19.5/14.8 | 21.9/27.4/23.2/19.1/14.2 |
ABS/short/middle/long/very long | concat | 15.4/17.1/16.4/13.6/14.9 | 13.4/13.4/14.5/13.5/11.9 | 14.5/13.1/16.7/13.6/13.3 | 14.8/12.5/15.6/14.3/15.8 | 15.9/22.2/17.1/12.5/14.4 |
ABS/short/middle/long/very long | parallel | 26.0/32.0/33.0/23.6/15.5 | 22.8/22.2/28.7/22.9/14.7 | 25.6/26.8/33.9/24.5/14.7 | 24.1/24.9/30.7/23.8/14.7 | 26.0/30.3/34.1/23.9/14.6 |
注:
- 考虑到跨场景评估时间非常长且性能较低,因此暂时不支持。上述指标是针对单场景(Intra-scenario)的。
concat
是Grounding DINO的默认推理模式,它将多个子句用点(.
)连接起来形成一个单独的句子进行推理。另一方面,“parallel”模式则在for循环中对每个子句分别进行推理。- MM-GDINO-T的配置文件是concat_dod:dod/grounding_dino_swin-t_pretrain_zeroshot_concat_dod.py和parallel_dod:dod/grounding_dino_swin-t_pretrain_zeroshot_parallel_dod.py
Pretrain Flickr30k Results
Model | Pre-Train Data | Val R@1 | Val R@5 | Val R@10 | Test R@1 | Test R@5 | Test R@10 |
---|---|---|---|---|---|---|---|
GLIP-T | O365,GoldG | 84.9 | 94.9 | 96.3 | 85.6 | 95.4 | 96.7 |
GLIP-T | O365,GoldG,CC3M,SBU | 85.3 | 95.5 | 96.9 | 86.0 | 95.9 | 97.2 |
GDINO-T | O365,GoldG,Cap4M | 87.8 | 96.6 | 98.0 | 88.1 | 96.9 | 98.2 |
MM-GDINO-T | O365,GoldG | 85.5 | 95.6 | 97.2 | 86.2 | 95.7 | 97.4 |
MM-GDINO-T | O365,GoldG,GRIT | 86.7 | 95.8 | 97.6 | 87.0 | 96.2 | 97.7 |
MM-GDINO-T | O365,GoldG,V3Det | 85.9 | 95.7 | 97.4 | 86.3 | 95.7 | 97.4 |
MM-GDINO-T | O365,GoldG,GRIT,V3Det | 86.7 | 96.0 | 97.6 | 87.2 | 96.2 | 97.7 |
注:
@1,5,10
指的是在预测的排名列表中,前1、5和10个位置的精确度。- MM-GDINO-T的配置文件位于:flickr30k/grounding_dino_swin-t-pretrain_flickr30k.py
通过微调验证预训练模型的泛化能力
RTTS
Architecture | Backbone | Lr schd | box AP |
---|---|---|---|
Faster R-CNN | R-50 | 1x | 48.1 |
Cascade R-CNN | R-50 | 1x | 50.8 |
ATSS | R-50 | 1x | 48.2 |
TOOD | R-50 | 1X | 50.8 |
MM-GDINO(zero-shot) | Swin-T | 49.8 | |
MM-GDINO | Swin-T | 1x | 69.1 |
- 参考指标来自 https://github.com/BIGWangYuDong/lqit/tree/main/configs/detection/rtts_dataset
- MM-GDINO-T 配置文件是:rtts/grounding_dino_swin-t_finetune_8xb4_1x_rtts.py
RUOD
Architecture | Backbone | Lr schd | box AP |
---|---|---|---|
Faster R-CNN | R-50 | 1x | 52.4 |
Cascade R-CNN | R-50 | 1x | 55.3 |
ATSS | R-50 | 1x | 55.7 |
TOOD | R-50 | 1X | 57.4 |
MM-GDINO(zero-shot) | Swin-T | 29.8 | |
MM-GDINO | Swin-T | 1x | 65.5 |
- 参考指标来自 https://github.com/BIGWangYuDong/lqit/tree/main/configs/detection/ruod_dataset
- MM-GDINO-T 配置文件位于:ruod/grounding_dino_swin-t_finetune_8xb4_1x_ruod.py
Brain Tumor
Architecture | Backbone | Lr schd | box AP |
---|---|---|---|
Faster R-CNN | R-50 | 50e | 43.5 |
Cascade R-CNN | R-50 | 50e | 46.2 |
DINO | R-50 | 50e | 46.4 |
Cascade-DINO | R-50 | 50e | 48.6 |
MM-GDINO | Swin-T | 50e | 47.5 |
- 参考指标来自 https://arxiv.org/abs/2307.11035
- MM-GDINO-T 配置文件是:brain_tumor/grounding_dino_swin-t_finetune_8xb4_50e_brain_tumor.py
Cityscapes
Architecture | Backbone | Lr schd | box AP |
---|---|---|---|
Faster R-CNN | R-50 | 50e | 30.1 |
Cascade R-CNN | R-50 | 50e | 31.8 |
DINO | R-50 | 50e | 34.5 |
Cascade-DINO | R-50 | 50e | 34.8 |
MM-GDINO(zero-shot) | Swin-T | 34.2 | |
MM-GDINO | Swin-T | 50e | 51.5 |
- 参考指标来自 https://arxiv.org/abs/2307.11035
- MM-GDINO-T 配置文件是:cityscapes/grounding_dino_swin-t_finetune_8xb4_50e_cityscapes.py
People in Painting
Architecture | Backbone | Lr schd | box AP |
---|---|---|---|
Faster R-CNN | R-50 | 50e | 17.0 |
Cascade R-CNN | R-50 | 50e | 18.0 |
DINO | R-50 | 50e | 12.0 |
Cascade-DINO | R-50 | 50e | 13.4 |
MM-GDINO(zero-shot) | Swin-T | 23.1 | |
MM-GDINO | Swin-T | 50e | 38.9 |
- 参考指标来自 https://arxiv.org/abs/2307.11035
- MM-GDINO-T 配置文件是 :people_in_painting/grounding_dino_swin-t_finetune_8xb4_50e_people_in_painting.py
COCO
(1) Closed-set performance
Architecture | Backbone | Lr schd | box AP |
---|---|---|---|
Faster R-CNN | R-50 | 1x | 37.4 |
Cascade R-CNN | R-50 | 1x | 40.3 |
ATSS | R-50 | 1x | 39.4 |
TOOD | R-50 | 1X | 42.4 |
DINO | R-50 | 1X | 50.1 |
GLIP(zero-shot) | Swin-T | 46.6 | |
GDINO(zero-shot) | Swin-T | 48.5 | |
MM-GDINO(zero-shot) | Swin-T | 50.4 | |
GLIP | Swin-T | 1x | 55.4 |
GDINO | Swin-T | 1x | 58.1 |
MM-GDINO | Swin-T | 1x | 58.2 |
- MM-GDINO-T 配置文件是:coco/grounding_dino_swin-t_finetune_16xb4_1x_coco.py
(2) 开放集继续预训练性能
Architecture | Backbone | Lr schd | box AP |
---|---|---|---|
GLIP(zero-shot) | Swin-T | 46.7 | |
GDINO(zero-shot) | Swin-T | 48.5 | |
MM-GDINO(zero-shot) | Swin-T | 50.4 | |
MM-GDINO | Swin-T | 1x | 54.7 |
- MM-GDINO-T 配置文件是 coco/grounding_dino_swin-t_finetune_16xb4_1x_sft_coco.py
- 由于COCO数据集的大小较小,仅在COCO上进行继续预训练很容易导致过拟合。上面显示的结果是来自第三个训练周期。我不推荐使用这种方法进行训练。
(3) 开放词汇性能
Architecture | Backbone | Lr schd | box AP | Base box AP | Novel box AP | box AP@50 | Base box AP@50 | Novel box AP@50 |
---|---|---|---|---|---|---|---|---|
MM-GDINO(zero-shot) | Swin-T | 51.1 | 48.4 | 58.9 | 66.7 | 64.0 | 74.2 | |
MM-GDINO | Swin-T | 1x | 57.2 | 56.1 | 60.4 | 73.6 | 73.0 | 75.3 |
- MM-GDINO-T 配置文件:coco/grounding_dino_swin-t_finetune_16xb4_1x_coco_48_17.py
LVIS 1.0
(1) 开放集继续预训练性能
Architecture | Backbone | Lr schd | MiniVal APr | MiniVal APc | MiniVal APf | MiniVal AP | Val1.0 APr | Val1.0 APc | Val1.0 APf | Val1.0 AP |
---|---|---|---|---|---|---|---|---|---|---|
GLIP(zero-shot) | Swin-T | 18.1 | 21.2 | 33.1 | 26.7 | 10.8 | 14.7 | 29.0 | 19.6 | |
GDINO(zero-shot) | Swin-T | 18.8 | 24.2 | 34.7 | 28.8 | 10.1 | 15.3 | 29.9 | 20.1 | |
MM-GDINO(zero-shot) | Swin-T | 34.2 | 37.4 | 46.2 | 41.4 | 23.6 | 27.6 | 40.5 | 31.9 | |
MM-GDINO | Swin-T | 1x | 50.7 | 58.8 | 60.1 | 58.7 | 45.2 | 50.2 | 56.1 | 51.7 |
- MM-GDINO-T 配置文件:lvis/grounding_dino_swin-t_finetune_16xb4_1x_lvis.py
(2) 开放词汇性能
Architecture | Backbone | Lr schd | MiniVal APr | MiniVal APc | MiniVal APf | MiniVal AP |
---|---|---|---|---|---|---|
MM-GDINO(zero-shot) | Swin-T | 34.2 | 37.4 | 46.2 | 41.4 | |
MM-GDINO | Swin-T | 1x | 43.2 | 57.4 | 59.3 | 57.1 |
- MM-GDINO-T 配置文件:lvis/grounding_dino_swin-t_finetune_16xb4_1x_lvis_866_337.py
RefEXP
RefCOCO
Architecture | Backbone | Lr schd | val @1 | val @5 | val @10 | testA @1 | testA @5 | testA @10 | testB @1 | testB @5 | testB @10 |
---|---|---|---|---|---|---|---|---|---|---|---|
GDINO(zero-shot) | Swin-T | 50.8 | 89.5 | 94.9 | 57.5 | 91.3 | 95.6 | 45.0 | 86.5 | 92.9 | |
MM-GDINO(zero-shot) | Swin-T | 53.1 | 89.7 | 95.1 | 59.1 | 91.0 | 95.5 | 46.8 | 87.8 | 93.6 | |
GDINO | Swin-T | UNK | 89.2 | 91.9 | 86.0 | ||||||
MM-GDINO | Swin-T | 5e | 89.5 | 98.6 | 99.4 | 91.4 | 99.2 | 99.8 | 86.6 | 97.9 | 99.1 |
- MM-GDINO-T 配置文件:refcoco/grounding_dino_swin-t_finetune_8xb4_5e_refcoco.py
RefCOCO+
Architecture | Backbone | Lr schd | val @1 | val @5 | val @10 | testA @1 | testA @5 | testA @10 | testB @1 | testB @5 | testB @10 |
---|---|---|---|---|---|---|---|---|---|---|---|
GDINO(zero-shot) | Swin-T | 51.6 | 86.4 | 92.6 | 57.3 | 86.7 | 92.7 | 46.4 | 84.1 | 90.7 | |
MM-GDINO(zero-shot) | Swin-T | 52.7 | 87.7 | 93.5 | 58.7 | 87.2 | 93.1 | 48.4 | 85.8 | 92.1 | |
GDINO | Swin-T | UNK | 81.1 | 87.4 | 74.7 | ||||||
MM-GDINO | Swin-T | 5e | 82.1 | 97.8 | 99.2 | 87.5 | 99.2 | 99.7 | 74.0 | 96.3 | 96.4 |
- MM-GDINO-T 配置文件:refcoco/grounding_dino_swin-t_finetune_8xb4_5e_refcoco_plus.py
RefCOCOg
Architecture | Backbone | Lr schd | val @1 | val @5 | val @10 | test @1 | test @5 | test @10 |
---|---|---|---|---|---|---|---|---|
GDINO(zero-shot) | Swin-T | 60.4 | 92.1 | 96.2 | 59.7 | 92.1 | 96.3 | |
MM-GDINO(zero-shot) | Swin-T | 62.9 | 93.3 | 97.2 | 62.9 | 93.9 | 97.4 | |
GDINO | Swin-T | UNK | 84.2 | 84.9 | ||||
MM-GDINO | Swin-T | 5e | 85.5 | 98.4 | 99.4 | 85.8 | 98.6 | 99.4 |
- MM-GDINO-T 配置文件:refcoco/grounding_dino_swin-t_finetune_8xb4_5e_refcocog.py
gRefCOCO
Architecture | Backbone | Lr schd | val Pr@(F1=1, IoU≥0.5) | val N-acc | testA Pr@(F1=1, IoU≥0.5) | testA N-acc | testB Pr@(F1=1, IoU≥0.5) | testB N-acc |
---|---|---|---|---|---|---|---|---|
GDINO(zero-shot) | Swin-T | 41.3 | 91.8 | 27.2 | 90.2 | 29.7 | 93.5 | |
MM-GDINO(zero-shot) | Swin-T | 41.0 | 91.3 | 26.1 | 93.0 | 30.4 | 92.3 | |
MM-GDINO | Swin-T | 5e | 45.1 | 64.7 | 42.5 | 65.5 | 40.3 | 63.2 |
- MM-GDINO-T 配置文件:refcoco/grounding_dino_swin-t_finetune_8xb4_5e_grefcoco.py
MM-GDINO-T 预训练数据准备和处理
MM-GDINO-T 模型中我们一共提供了 5 种不同数据组合的预训练配置,数据采用逐步累加的方式进行训练,因此用户可以根据自己的实际需求准备数据。
用到的数据集
1 Objects365 v1
对应的训练配置为:./grounding_dino_swin-t_pretrain_obj365.py
Objects365_v1 可以从https://opendatalab.com/OpenDataLab/Objects365_v1
下载,其提供了 CLI 和 SDK 两者下载方式。
下载并解压后,将其放置或者软链接到 data/objects365v1
目录下,目录结构如下:
mmdetection
├── configs
├── data
│ ├── objects365v1
│ │ ├── objects365_train.json
│ │ ├── objects365_val.json
│ │ ├── train
│ │ │ ├── xxx.jpg
│ │ │ ├── ...
│ │ ├── val
│ │ │ ├── xxxx.jpg
│ │ │ ├── ...
│ │ ├── test
然后使用 coco2odvg.py 转换为训练所需的 ODVG 格式:
python tools/dataset_converters/coco2odvg.py data/objects365v1/objects365_train.json -d o365v1
程序运行完成后会在 data/objects365v1
目录下创建 o365v1_train_od.json
和 o365v1_label_map.json
两个新文件,完整结构如下:
mmdetection
├── configs
├── data
│ ├── objects365v1
│ │ ├── objects365_train.json
│ │ ├── objects365_val.json
│ │ ├── o365v1_train_od.json
│ │ ├── o365v1_label_map.json
│ │ ├── train
│ │ │ ├── xxx.jpg
│ │ │ ├── ...
│ │ ├── val
│ │ │ ├── xxxx.jpg
│ │ │ ├── ...
│ │ ├── test
2 COCO 2017
上述配置在训练过程中会评估 COCO 2017 数据集的性能,因此需要准备 COCO 2017 数据集。你可以从 COCO 官网下载或者从 opendatalab 下载
下载并解压后,将其放置或者软链接到 data/coco
目录下,目录结构如下:
mmdetection
├── configs
├── data
│ ├── coco
│ │ ├── annotations
│ │ │ ├── instances_train2017.json
│ │ │ ├── instances_val2017.json
│ │ ├── train2017
│ │ │ ├── xxx.jpg
│ │ │ ├── ...
│ │ ├── val2017
│ │ │ ├── xxxx.jpg
│ │ │ ├── ...
3 GoldG
下载该数据集后就可以训练 :./grounding_dino_swin-t_pretrain_obj365_goldg.py配置了。
GoldG 数据集包括 GQA
和 Flickr30k
两个数据集,来自 GLIP 论文中提到的 MixedGrounding 数据集,其排除了 COCO 数据集。下载链接为 mdetr_annotations,我们目前需要的是 mdetr_annotations/final_mixed_train_no_coco.json
和 mdetr_annotations/final_flickr_separateGT_train.json
文件。
然后下载 GQA images 图片。下载并解压后,将其放置或者软链接到 data/gqa
目录下,目录结构如下:
mmdetection
├── configs
├── data
│ ├── gqa
| | ├── final_mixed_train_no_coco.json
│ │ ├── images
│ │ │ ├── xxx.jpg
│ │ │ ├── ...
然后下载 Flickr30k images 图片。这个数据下载需要先申请,再获得下载链接后才可以下载。下载并解压后,将其放置或者软链接到 data/flickr30k_entities
目录下,目录结构如下:
mmdetection
├── configs
├── data
│ ├── flickr30k_entities
│ │ ├── final_flickr_separateGT_train.json
│ │ ├── flickr30k_images
│ │ │ ├── xxx.jpg
│ │ │ ├── ...
对于 GQA 数据集,你需要使用 goldg2odvg.py 转换为训练所需的 ODVG 格式:
python tools/dataset_converters/goldg2odvg.py data/gqa/final_mixed_train_no_coco.json
程序运行完成后会在 data/gqa
目录下创建 final_mixed_train_no_coco_vg.json
新文件,完整结构如下:
mmdetection
├── configs
├── data
│ ├── gqa
| | ├── final_mixed_train_no_coco.json
| | ├── final_mixed_train_no_coco_vg.json
│ │ ├── images
│ │ │ ├── xxx.jpg
│ │ │ ├── ...
对于 Flickr30k 数据集,你需要使用 goldg2odvg.py 转换为训练所需的 ODVG 格式:
python tools/dataset_converters/goldg2odvg.py data/flickr30k_entities/final_flickr_separateGT_train.json
程序运行完成后会在 data/flickr30k_entities
目录下创建 final_flickr_separateGT_train_vg.json
新文件,完整结构如下:
mmdetection
├── configs
├── data
│ ├── flickr30k_entities
│ │ ├── final_flickr_separateGT_train.json
│ │ ├── final_flickr_separateGT_train_vg.json
│ │ ├── flickr30k_images
│ │ │ ├── xxx.jpg
│ │ │ ├── ...
4 GRIT-20M
对应的训练配置为 grounding_dino_swin-t_pretrain_obj365_goldg_grit9m
GRIT数据集可以从 GRIT 中使用 img2dataset 包下载,默认指令下载后数据集大小为 1.1T,下载和处理预估需要至少 2T 硬盘空间,可根据硬盘容量酌情下载。下载后原始格式为:
mmdetection
├── configs
├── data
│ ├── grit_raw
│ │ ├── 00000_stats.json
│ │ ├── 00000.parquet
│ │ ├── 00000.tar
│ │ ├── 00001_stats.json
│ │ ├── 00001.parquet
│ │ ├── 00001.tar
│ │ ├── ...
下载后需要对格式进行进一步处理:
python tools/dataset_converters/grit_processing.py data/grit_raw data/grit_processed
处理后的格式为:
mmdetection
├── configs
├── data
│ ├── grit_processed
│ │ ├── annotations
│ │ │ ├── 00000.json
│ │ │ ├── 00001.json
│ │ │ ├── ...
│ │ ├── images
│ │ │ ├── 00000
│ │ │ │ ├── 000000000.jpg
│ │ │ │ ├── 000000003.jpg
│ │ │ │ ├── 000000004.jpg
│ │ │ │ ├── ...
│ │ │ ├── 00001
│ │ │ ├── ...
对于 GRIT 数据集,你需要使用 grit2odvg.py 转化成需要的 ODVG 格式:
python tools/dataset_converters/grit2odvg.py data/grit_processed/
程序运行完成后会在 data/grit_processed
目录下创建 grit20m_vg.json
新文件,大概包含 9M 条数据,完整结构如下:
mmdetection
├── configs
├── data
│ ├── grit_processed
| | ├── grit20m_vg.json
│ │ ├── annotations
│ │ │ ├── 00000.json
│ │ │ ├── 00001.json
│ │ │ ├── ...
│ │ ├── images
│ │ │ ├── 00000
│ │ │ │ ├── 000000000.jpg
│ │ │ │ ├── 000000003.jpg
│ │ │ │ ├── 000000004.jpg
│ │ │ │ ├── ...
│ │ │ ├── 00001
│ │ │ ├── ...
5 V3Det
对应的训练配置为
- grounding_dino_swin-t_pretrain_obj365_goldg_v3det
- grounding_dino_swin-t_pretrain_obj365_goldg_grit9m_v3det
V3Det 数据集下载可以从 opendatalab 下载,下载并解压后,将其放置或者软链接到 data/v3det
目录下,目录结构如下:
mmdetection
├── configs
├── data
│ ├── v3det
│ │ ├── annotations
│ │ | ├── v3det_2023_v1_train.json
│ │ ├── images
│ │ │ ├── a00000066
│ │ │ │ ├── xxx.jpg
│ │ │ ├── ...
然后使用 coco2odvg.py 转换为训练所需的 ODVG 格式:
python tools/dataset_converters/coco2odvg.py data/v3det/annotations/v3det_2023_v1_train.json -d v3det
程序运行完成后会在 data/v3det/annotations
目录下创建目录下创建 v3det_2023_v1_train_od.json
和 v3det_2023_v1_label_map.json
两个新文件,完整结构如下:
mmdetection
├── configs
├── data
│ ├── v3det
│ │ ├── annotations
│ │ | ├── v3det_2023_v1_train.json
│ │ | ├── v3det_2023_v1_train_od.json
│ │ | ├── v3det_2023_v1_label_map.json
│ │ ├── images
│ │ │ ├── a00000066
│ │ │ │ ├── xxx.jpg
│ │ │ ├── ...
6 数据切分和可视化
考虑到用户需要准备的数据集过多,不方便对图片和标注进行训练前确认,因此我们提供了一个数据切分和可视化的工具,可以将数据集切分为 tiny 版本,然后使用可视化脚本查看图片和标签正确性。
- 切分数据集
脚本位于 这里, 以 Object365 v1
为例,切分数据集的命令如下:
python tools/misc/split_odvg.py data/object365_v1/ o365v1_train_od.json train your_output_dir --label-map-file o365v1_label_map.json -n 200
上述脚本运行后会在 your_output_dir
目录下创建和 data/object365_v1/
一样的文件夹结构,但是只会保存 200 张训练图片和对应的 json,方便用户查看。
- 可视化原始数据集
脚本位于 这里, 以 Object365 v1
为例,可视化数据集的命令如下:
python tools/analysis_tools/browse_grounding_raw.py data/object365_v1/ o365v1_train_od.json train --label-map-file o365v1_label_map.json -o your_output_dir --not-show
上述脚本运行后会在 your_output_dir
目录下生成同时包括图片和标签的图片,方便用户查看。
- 可视化 dataset 输出的数据集
脚本位于 这里, 用户可以通过该脚本查看 dataset 输出的结果即包括了数据增强的结果。 以 Object365 v1
为例,可视化数据集的命令如下:
python tools/analysis_tools/browse_grounding_dataset.py configs/mm_grounding_dino/grounding_dino_swin-t_pretrain_obj365.py -o your_output_dir --not-show
上述脚本运行后会在 your_output_dir
目录下生成同时包括图片和标签的图片,方便用户查看。
MM-GDINO-L 预训练数据准备和处理
用到的数据集
1 Object365 v2
Objects365_v2 可以从 opendatalab 下载,其提供了 CLI 和 SDK 两者下载方式。
下载并解压后,将其放置或者软链接到 data/objects365v2
目录下,目录结构如下:
mmdetection
├── configs
├── data
│ ├── objects365v2
│ │ ├── annotations
│ │ │ ├── zhiyuan_objv2_train.json
│ │ ├── train
│ │ │ ├── patch0
│ │ │ │ ├── xxx.jpg
│ │ │ ├── ...
由于 objects365v2 类别中有部分类名是错误的,因此需要先进行修正。
python tools/dataset_converters/fix_o365_names.py
会在 data/objects365v2/annotations
下生成新的标注文件 zhiyuan_objv2_train_fixname.json
。
然后使用 coco2odvg.py 转换为训练所需的 ODVG 格式:
python tools/dataset_converters/coco2odvg.py data/objects365v2/annotations/zhiyuan_objv2_train_fixname.json -d o365v2
程序运行完成后会在 data/objects365v2
目录下创建 zhiyuan_objv2_train_fixname_od.json
和 o365v2_label_map.json
两个新文件,完整结构如下:
mmdetection
├── configs
├── data
│ ├── objects365v2
│ │ ├── annotations
│ │ │ ├── zhiyuan_objv2_train.json
│ │ │ ├── zhiyuan_objv2_train_fixname.json
│ │ │ ├── zhiyuan_objv2_train_fixname_od.json
│ │ │ ├── o365v2_label_map.json
│ │ ├── train
│ │ │ ├── patch0
│ │ │ │ ├── xxx.jpg
│ │ │ ├── ...
2 OpenImages v6
OpenImages v6 可以从 官网 下载,由于数据集比较大,需要花费一定的时间,下载完成后文件结构如下:
mmdetection
├── configs
├── data
│ ├── OpenImages
│ │ ├── annotations
| │ │ ├── oidv6-train-annotations-bbox.csv
| │ │ ├── class-descriptions-boxable.csv
│ │ ├── OpenImages
│ │ │ ├── train
│ │ │ │ ├── xxx.jpg
│ │ │ ├── ...
然后使用 openimages2odvg.py 转换为训练所需的 ODVG 格式:
python tools/dataset_converters/openimages2odvg.py data/OpenImages/annotations
程序运行完成后会在 data/OpenImages/annotations
目录下创建 oidv6-train-annotation_od.json
和 openimages_label_map.json
两个新文件,完整结构如下:
mmdetection
├── configs
├── data
│ ├── OpenImages
│ │ ├── annotations
| │ │ ├── oidv6-train-annotations-bbox.csv
| │ │ ├── class-descriptions-boxable.csv
| │ │ ├── oidv6-train-annotations_od.json
| │ │ ├── openimages_label_map.json
│ │ ├── OpenImages
│ │ │ ├── train
│ │ │ │ ├── xxx.jpg
│ │ │ ├── ...
3 V3Det
参见前面的 MM-GDINO-T 预训练数据准备和处理 数据准备部分,完整数据集结构如下:
mmdetection
├── configs
├── data
│ ├── v3det
│ │ ├── annotations
│ │ | ├── v3det_2023_v1_train.json
│ │ | ├── v3det_2023_v1_train_od.json
│ │ | ├── v3det_2023_v1_label_map.json
│ │ ├── images
│ │ │ ├── a00000066
│ │ │ │ ├── xxx.jpg
│ │ │ ├── ...
4 LVIS 1.0
参见后面的 微调数据集准备
的 2 LVIS 1.0
部分。完整数据集结构如下:
mmdetection
├── configs
├── data
│ ├── coco
│ │ ├── annotations
│ │ │ ├── instances_train2017.json
│ │ │ ├── lvis_v1_train.json
│ │ │ ├── lvis_v1_val.json
│ │ │ ├── lvis_v1_train_od.json
│ │ │ ├── lvis_v1_label_map.json
│ │ │ ├── instances_val2017.json
│ │ │ ├── lvis_v1_minival_inserted_image_name.json
│ │ │ ├── lvis_od_val.json
│ │ ├── train2017
│ │ │ ├── xxx.jpg
│ │ │ ├── ...
│ │ ├── val2017
│ │ │ ├── xxxx.jpg
│ │ │ ├── ...
5 COCO2017 OD
数据准备可以参考前面的 MM-GDINO-T 预训练数据准备和处理
部分。为了方便后续处理,请将下载的 mdetr_annotations 文件夹软链接或者移动到 data/coco
路径下
完整数据集结构如下:
mmdetection
├── configs
├── data
│ ├── coco
│ │ ├── annotations
│ │ │ ├── instances_train2017.json
│ │ │ ├── instances_val2017.json
│ │ ├── mdetr_annotations
│ │ │ ├── final_refexp_val.json
│ │ │ ├── finetune_refcoco_testA.json
│ │ │ ├── ...
│ │ ├── train2017
│ │ │ ├── xxx.jpg
│ │ │ ├── ...
│ │ ├── val2017
│ │ │ ├── xxxx.jpg
│ │ │ ├── ...
由于 COCO2017 train 和 RefCOCO/RefCOCO+/RefCOCOg/gRefCOCO val 中存在部分重叠,如果不提前移除,在评测 RefExp 时候会存在数据泄露。
python tools/dataset_converters/remove_cocotrain2017_from_refcoco.py data/coco/mdetr_annotations data/coco/annotations/instances_train2017.json
会在 data/coco/annotations
目录下创建 instances_train2017_norefval.json
新文件。最后使用 coco2odvg.py 转换为训练所需的 ODVG 格式:
python tools/dataset_converters/coco2odvg.py data/coco/annotations/instances_train2017_norefval.json -d coco
会在 data/coco/annotations
目录下创建 instances_train2017_norefval_od.json
和 coco_label_map.json
两个新文件,完整结构如下:
mmdetection
├── configs
├── data
│ ├── coco
│ │ ├── annotations
│ │ │ ├── instances_train2017.json
│ │ │ ├── instances_val2017.json
│ │ │ ├── instances_train2017_norefval_od.json
│ │ │ ├── coco_label_map.json
│ │ ├── mdetr_annotations
│ │ │ ├── final_refexp_val.json
│ │ │ ├── finetune_refcoco_testA.json
│ │ │ ├── ...
│ │ ├── train2017
│ │ │ ├── xxx.jpg
│ │ │ ├── ...
│ │ ├── val2017
│ │ │ ├── xxxx.jpg
│ │ │ ├── ...
注意: COCO2017 train 和 LVIS 1.0 val 数据集有 15000 张图片重复,因此一旦在训练中使用了 COCO2017 train,那么 LVIS 1.0 val 的评测结果就存在数据泄露问题,LVIS 1.0 minival 没有这个问题。
6 GoldG
参见 MM-GDINO-T 预训练数据准备和处理 部分
mmdetection
├── configs
├── data
│ ├── flickr30k_entities
│ │ ├── final_flickr_separateGT_train.json
│ │ ├── final_flickr_separateGT_train_vg.json
│ │ ├── flickr30k_images
│ │ │ ├── xxx.jpg
│ │ │ ├── ...
│ ├── gqa
| | ├── final_mixed_train_no_coco.json
| | ├── final_mixed_train_no_coco_vg.json
│ │ ├── images
│ │ │ ├── xxx.jpg
│ │ │ ├── ...
7 COCO2014 VG
MDetr 中提供了 COCO2014 train 的 Phrase Grounding 版本标注, 最原始标注文件为 final_mixed_train.json
,和之前类似,文件结构如下:
mmdetection
├── configs
├── data
│ ├── coco
│ │ ├── annotations
│ │ │ ├── instances_train2017.json
│ │ │ ├── instances_val2017.json
│ │ ├── mdetr_annotations
│ │ │ ├── final_mixed_train.json
│ │ │ ├── ...
│ │ ├── train2017
│ │ │ ├── xxx.jpg
│ │ │ ├── ...
│ │ ├── train2014
│ │ │ ├── xxx.jpg
│ │ │ ├── ...
我们可以从 final_mixed_train.json
中提取出 COCO 部分数据
python tools/dataset_converters/extract_coco_from_mixed.py data/coco/mdetr_annotations/final_mixed_train.json
会在 data/coco/mdetr_annotations
目录下创建 final_mixed_train_only_coco.json
新文件,最后使用 goldg2odvg.py 转换为训练所需的 ODVG 格式:
python tools/dataset_converters/goldg2odvg.py data/coco/mdetr_annotations/final_mixed_train_only_coco.json
会在 data/coco/mdetr_annotations
目录下创建 final_mixed_train_only_coco_vg.json
新文件,完整结构如下:
mmdetection
├── configs
├── data
│ ├── coco
│ │ ├── annotations
│ │ │ ├── instances_train2017.json
│ │ │ ├── instances_val2017.json
│ │ ├── mdetr_annotations
│ │ │ ├── final_mixed_train.json
│ │ │ ├── final_mixed_train_only_coco.json
│ │ │ ├── final_mixed_train_only_coco_vg.json
│ │ │ ├── ...
│ │ ├── train2017
│ │ │ ├── xxx.jpg
│ │ │ ├── ...
│ │ ├── train2014
│ │ │ ├── xxx.jpg
│ │ │ ├── ...
注意: COCO2014 train 和 COCO2017 val 没有重复图片,因此不用担心 COCO 评测的数据泄露问题。
8 Referring Expression Comprehension
其一共包括 4 个数据集。数据准备部分请参见 微调数据集准备 部分。
mmdetection
├── configs
├── data
│ ├── coco
│ │ ├── annotations
│ │ │ ├── instances_train2017.json
│ │ │ ├── instances_val2017.json
│ │ │ ├── instances_train2014.json
│ │ ├── train2017
│ │ │ ├── xxx.jpg
│ │ │ ├── ...
│ │ ├── val2017
│ │ │ ├── xxxx.jpg
│ │ │ ├── ...
│ │ ├── train2014
│ │ │ ├── xxx.jpg
│ │ │ ├── ...
│ │ ├── mdetr_annotations
│ │ │ ├── final_refexp_val.json
│ │ │ ├── finetune_refcoco_testA.json
│ │ │ ├── finetune_refcoco_testB.json
│ │ │ ├── finetune_refcoco+_testA.json
│ │ │ ├── finetune_refcoco+_testB.json
│ │ │ ├── finetune_refcocog_test.json
│ │ │ ├── finetune_refcoco_train_vg.json
│ │ │ ├── finetune_refcoco+_train_vg.json
│ │ │ ├── finetune_refcocog_train_vg.json
│ │ │ ├── finetune_grefcoco_train_vg.json
9 GRIT-20M
参见 MM-GDINO-T 预训练数据准备和处理 部分
评测数据集准备
1 COCO 2017
数据准备流程和前面描述一致,最终结构如下:
mmdetection
├── configs
├── data
│ ├── coco
│ │ ├── annotations
│ │ │ ├── instances_train2017.json
│ │ │ ├── instances_val2017.json
│ │ ├── train2017
│ │ │ ├── xxx.jpg
│ │ │ ├── ...
│ │ ├── val2017
│ │ │ ├── xxxx.jpg
│ │ │ ├── ...
2 LVIS 1.0
LVIS 1.0 val 数据集包括 mini 和全量两个版本,mini 版本存在的意义是:
- LVIS val 全量评测数据集比较大,评测一次需要比较久的时间
- LVIS val 全量数据集中包括了 15000 张 COCO2017 train, 如果用户使用了 COCO2017 数据进行训练,那么将存在数据泄露问题
LVIS 1.0 图片和 COCO2017 数据集图片完全一样,只是提供了新的标注而已,minival 标注文件可以从 这里下载, val 1.0 标注文件可以从 这里 下载。 最终结构如下:
mmdetection
├── configs
├── data
│ ├── coco
│ │ ├── annotations
│ │ │ ├── instances_train2017.json
│ │ │ ├── instances_val2017.json
│ │ │ ├── lvis_v1_minival_inserted_image_name.json
│ │ │ ├── lvis_od_val.json
│ │ ├── train2017
│ │ │ ├── xxx.jpg
│ │ │ ├── ...
│ │ ├── val2017
│ │ │ ├── xxxx.jpg
│ │ │ ├── ...
3 ODinW
ODinw 全称为 Object Detection in the Wild,是用于验证 grounding 预训练模型在不同实际场景中的泛化能力的数据集,其包括两个子集,分别是 ODinW13 和 ODinW35,代表是由 13 和 35 个数据集组成的。你可以从 这里下载,然后对每个文件进行解压,最终结构如下:
mmdetection
├── configs
├── data
│ ├── odinw
│ │ ├── AerialMaritimeDrone
│ │ | |── large
│ │ | | ├── test
│ │ | | ├── train
│ │ | | ├── valid
│ │ | |── tiled
│ │ ├── AmericanSignLanguageLetters
│ │ ├── Aquarium
│ │ ├── BCCD
│ │ ├── ...
在评测 ODinW3535 时候由于需要自定义 prompt,因此需要提前对标注的 json 文件进行处理,你可以使用 override_category.py 脚本进行处理,处理后会生成新的标注文件,不会覆盖原先的标注文件。
python configs/mm_grounding_dino/odinw/override_category.py data/odinw/
4 DOD
DOD 来自 Described Object Detection: Liberating Object Detection with Flexible Expressions。其数据集可以从 这里下载,最终的数据集结构如下:
mmdetection
├── configs
├── data
│ ├── d3
│ │ ├── d3_images
│ │ ├── d3_json
│ │ ├── d3_pkl
5 Flickr30k Entities
在前面 GoldG 数据准备章节中我们已经下载了 Flickr30k 训练所需文件,评估所需的文件是 2 个 json 文件,你可以从 这里 和 这里下载,最终的数据集结构如下:
mmdetection
├── configs
├── data
│ ├── flickr30k_entities
│ │ ├── final_flickr_separateGT_train.json
│ │ ├── final_flickr_separateGT_val.json
│ │ ├── final_flickr_separateGT_test.json
│ │ ├── final_flickr_separateGT_train_vg.json
│ │ ├── flickr30k_images
│ │ │ ├── xxx.jpg
│ │ │ ├── ...
6 Referring Expression Comprehension
指代性表达式理解包括 4 个数据集: RefCOCO, RefCOCO+, RefCOCOg, gRefCOCO。这 4 个数据集所采用的图片都来自于 COCO2014 train,和 COCO2017 类似,你可以从 COCO 官方或者 opendatalab 中下载,而标注可以直接从 这里 下载,mdetr_annotations 文件夹里面包括了其他大量的标注,你如果觉得数量过多,可以只下载所需要的几个 json 文件即可。最终的数据集结构如下:
mmdetection
├── configs
├── data
│ ├── coco
│ │ ├── annotations
│ │ │ ├── instances_train2017.json
│ │ │ ├── instances_val2017.json
│ │ │ ├── instances_train2014.json
│ │ ├── train2017
│ │ │ ├── xxx.jpg
│ │ │ ├── ...
│ │ ├── val2017
│ │ │ ├── xxxx.jpg
│ │ │ ├── ...
│ │ ├── train2014
│ │ │ ├── xxx.jpg
│ │ │ ├── ...
│ │ ├── mdetr_annotations
│ │ │ ├── final_refexp_val.json
│ │ │ ├── finetune_refcoco_testA.json
│ │ │ ├── finetune_refcoco_testB.json
│ │ │ ├── finetune_refcoco+_testA.json
│ │ │ ├── finetune_refcoco+_testB.json
│ │ │ ├── finetune_refcocog_test.json
│ │ │ ├── finetune_refcocog_test.json
注意 gRefCOCO 是在 GREC: Generalized Referring Expression Comprehension 被提出,并不在 mdetr_annotations
文件夹中,需要自行处理。具体步骤为:
- 下载 gRefCOCO,并解压到 data/coco/ 文件夹中
mmdetection
├── configs
├── data
│ ├── coco
│ │ ├── annotations
│ │ │ ├── instances_train2017.json
│ │ │ ├── instances_val2017.json
│ │ │ ├── instances_train2014.json
│ │ ├── train2017
│ │ │ ├── xxx.jpg
│ │ │ ├── ...
│ │ ├── val2017
│ │ │ ├── xxxx.jpg
│ │ │ ├── ...
│ │ ├── train2014
│ │ │ ├── xxx.jpg
│ │ │ ├── ...
│ │ ├── mdetr_annotations
│ │ ├── grefs
│ │ │ ├── grefs(unc).json
│ │ │ ├── instances.json
- 转换为 coco 格式
你可以使用 gRefCOCO 官方提供的转换脚本。注意需要将被注释的 161 行打开,并注释 160 行才可以得到全量的 json 文件。
# 需要克隆官方 repo
git clone https://github.com/henghuiding/gRefCOCO.git
cd gRefCOCO/mdetr
python scripts/fine-tuning/grefexp_coco_format.py --data_path ../../data/coco/grefs --out_path ../../data/coco/mdetr_annotations/ --coco_path ../../data/coco
会在 data/coco/mdetr_annotations/
文件夹中生成 4 个 json 文件,完整的数据集结构如下:
mmdetection
├── configs
├── data
│ ├── coco
│ │ ├── annotations
│ │ │ ├── instances_train2017.json
│ │ │ ├── instances_val2017.json
│ │ │ ├── instances_train2014.json
│ │ ├── train2017
│ │ │ ├── xxx.jpg
│ │ │ ├── ...
│ │ ├── val2017
│ │ │ ├── xxxx.jpg
│ │ │ ├── ...
│ │ ├── train2014
│ │ │ ├── xxx.jpg
│ │ │ ├── ...
│ │ ├── mdetr_annotations
│ │ │ ├── final_refexp_val.json
│ │ │ ├── finetune_refcoco_testA.json
│ │ │ ├── finetune_refcoco_testB.json
│ │ │ ├── finetune_grefcoco_train.json
│ │ │ ├── finetune_grefcoco_val.json
│ │ │ ├── finetune_grefcoco_testA.json
│ │ │ ├── finetune_grefcoco_testB.json
微调数据集准备
1 COCO 2017
COCO 是检测领域最常用的数据集,我们希望能够更充分探索其微调模式。从目前发展来看,一共有 3 种微调方式:
- 闭集微调,即微调后文本端将无法修改描述,转变为闭集算法,在 COCO 上性能能够最大化,但是失去了通用性。
- 开集继续预训练微调,即对 COCO 数据集采用和预训练一致的预训练手段。此时有两种做法,第一种是降低学习率并固定某些模块,仅仅在 COCO 数据上预训练,第二种是将 COCO 数据和部分预训练数据混合一起训练,两种方式的目的都是在尽可能不降低泛化性时提高 COCO 数据集性能
- 开放词汇微调,即采用 OVD 领域常用做法,将 COCO 类别分成 base 类和 novel 类,训练时候仅仅在 base 类上进行,评测在 base 和 novel 类上进行。这种方式可以验证 COCO OVD 能力,目的也是在尽可能不降低泛化性时提高 COCO 数据集性能
(1) 闭集微调
这个部分无需准备数据,直接用之前的数据即可。
mmdetection
├── configs
├── data
│ ├── coco
│ │ ├── annotations
│ │ │ ├── instances_train2017.json
│ │ │ ├── instances_val2017.json
│ │ ├── train2017
│ │ │ ├── xxx.jpg
│ │ │ ├── ...
│ │ ├── val2017
│ │ │ ├── xxxx.jpg
│ │ │ ├── ...
(2) 开集继续预训练微调
这种方式需要将 COCO 训练数据转换为 ODVG 格式,你可以使用如下命令转换:
python tools/dataset_converters/coco2odvg.py data/coco/annotations/instances_train2017.json -d coco
会在 data/coco/annotations/
下生成新的 instances_train2017_od.json
和 coco2017_label_map.json
,完整的数据集结构如下:
mmdetection
├── configs
├── data
│ ├── coco
│ │ ├── annotations
│ │ │ ├── instances_train2017.json
│ │ │ ├── instances_train2017_od.json
│ │ │ ├── coco2017_label_map.json
│ │ │ ├── instances_val2017.json
│ │ ├── train2017
│ │ │ ├── xxx.jpg
│ │ │ ├── ...
│ │ ├── val2017
│ │ │ ├── xxxx.jpg
│ │ │ ├── ...
在得到数据后,你可以自行选择单独预习还是混合预训练方式。
(3) 开放词汇微调
这种方式需要将 COCO 训练数据转换为 OVD 格式,你可以使用如下命令转换:
python tools/dataset_converters/coco2ovd.py data/coco/
会在 data/coco/annotations/
下生成新的 instances_val2017_all_2.json
和 instances_val2017_seen_2.json
,完整的数据集结构如下:
mmdetection
├── configs
├── data
│ ├── coco
│ │ ├── annotations
│ │ │ ├── instances_train2017.json
│ │ │ ├── instances_train2017_od.json
│ │ │ ├── instances_val2017_all_2.json
│ │ │ ├── instances_val2017_seen_2.json
│ │ │ ├── coco2017_label_map.json
│ │ │ ├── instances_val2017.json
│ │ ├── train2017
│ │ │ ├── xxx.jpg
│ │ │ ├── ...
│ │ ├── val2017
│ │ │ ├── xxxx.jpg
│ │ │ ├── ...
然后可以直接使用 配置 进行训练和测试。
2 LVIS 1.0
LVIS 是一个包括 1203 类的数据集,同时也是一个长尾联邦数据集,对其进行微调很有意义。 由于其类别过多,我们无法对其进行闭集微调,因此只能采用开集继续预训练微调和开放词汇微调。
你需要先准备好 LVIS 训练 JSON 文件,你可以从 这里 下载,我们只需要 lvis_v1_train.json
和 lvis_v1_val.json
,然后将其放到 data/coco/annotations/
下,然后运行如下命令:
mmdetection
├── configs
├── data
│ ├── coco
│ │ ├── annotations
│ │ │ ├── instances_train2017.json
│ │ │ ├── lvis_v1_train.json
│ │ │ ├── lvis_v1_val.json
│ │ │ ├── instances_val2017.json
│ │ │ ├── lvis_v1_minival_inserted_image_name.json
│ │ │ ├── lvis_od_val.json
│ │ ├── train2017
│ │ │ ├── xxx.jpg
│ │ │ ├── ...
│ │ ├── val2017
│ │ │ ├── xxxx.jpg
│ │ │ ├── ...
(1) 开集继续预训练微调
使用如下命令转换为 ODVG 格式:
python tools/dataset_converters/lvis2odvg.py data/coco/annotations/lvis_v1_train.json
会在 data/coco/annotations/
下生成新的 lvis_v1_train_od.json
和 lvis_v1_label_map.json
,完整的数据集结构如下:
mmdetection
├── configs
├── data
│ ├── coco
│ │ ├── annotations
│ │ │ ├── instances_train2017.json
│ │ │ ├── lvis_v1_train.json
│ │ │ ├── lvis_v1_val.json
│ │ │ ├── lvis_v1_train_od.json
│ │ │ ├── lvis_v1_label_map.json
│ │ │ ├── instances_val2017.json
│ │ │ ├── lvis_v1_minival_inserted_image_name.json
│ │ │ ├── lvis_od_val.json
│ │ ├── train2017
│ │ │ ├── xxx.jpg
│ │ │ ├── ...
│ │ ├── val2017
│ │ │ ├── xxxx.jpg
│ │ │ ├── ...
然后可以直接使用 配置 进行训练测试,或者你修改配置将其和部分预训练数据集混合使用。
(2) 开放词汇微调
使用如下命令转换为 OVD 格式:
python tools/dataset_converters/lvis2ovd.py data/coco/
会在 data/coco/annotations/
下生成新的 lvis_v1_train_od_norare.json
和 lvis_v1_label_map_norare.json
,完整的数据集结构如下:
mmdetection
├── configs
├── data
│ ├── coco
│ │ ├── annotations
│ │ │ ├── instances_train2017.json
│ │ │ ├── lvis_v1_train.json
│ │ │ ├── lvis_v1_val.json
│ │ │ ├── lvis_v1_train_od.json
│ │ │ ├── lvis_v1_label_map.json
│ │ │ ├── instances_val2017.json
│ │ │ ├── lvis_v1_minival_inserted_image_name.json
│ │ │ ├── lvis_od_val.json
│ │ │ ├── lvis_v1_train_od_norare.json
│ │ │ ├── lvis_v1_label_map_norare.json
│ │ ├── train2017
│ │ │ ├── xxx.jpg
│ │ │ ├── ...
│ │ ├── val2017
│ │ │ ├── xxxx.jpg
│ │ │ ├── ...
然后可以直接使用 配置 进行训练测试
3 RTTS
RTTS 是一个浓雾天气数据集,该数据集包含 4,322 张雾天图像,包含五个类:自行车 (bicycle)、公共汽车 (bus)、汽车 (car)、摩托车 (motorbike) 和人 (person)。可以从 这里下载, 然后解压到 data/RTTS/
文件夹中。完整的数据集结构如下:
mmdetection
├── configs
├── data
│ ├── RTTS
│ │ ├── annotations_json
│ │ ├── annotations_xml
│ │ ├── ImageSets
│ │ ├── JPEGImages
4 RUOD
RUOD 是一个水下目标检测数据集,你可以从 这里下载, 然后解压到 data/RUOD/
文件夹中。完整的数据集结构如下:
mmdetection
├── configs
├── data
│ ├── RUOD
│ │ ├── Environment_pic
│ │ ├── Environmet_ANN
│ │ ├── RUOD_ANN
│ │ ├── RUOD_pic
5 Brain Tumor
Brain Tumor 是一个医学领域的 2d 检测数据集,你可以从 这里下载, 请注意选择 COCO JSON
格式。然后解压到 data/brain_tumor_v2/
文件夹中。完整的数据集结构如下:
mmdetection
├── configs
├── data
│ ├── brain_tumor_v2
│ │ ├── test
│ │ ├── train
│ │ ├── valid
6 Cityscapes
Cityscapes 是一个城市街景数据集,你可以从 这里 或者 opendatalab 中下载, 然后解压到 data/cityscapes/
文件夹中。完整的数据集结构如下:
mmdetection
├── configs
├── data
│ ├── cityscapes
│ │ ├── annotations
│ │ ├── leftImg8bit
│ │ │ ├── train
│ │ │ ├── val
│ │ ├── gtFine
│ │ │ ├── train
│ │ │ ├── val
在下载后,然后使用 cityscapes.py 脚本生成我们所需要的 json 格式
python tools/dataset_converters/cityscapes.py data/cityscapes/
会在 annotations 中生成 3 个新的 json 文件。完整的数据集结构如下:
mmdetection
├── configs
├── data
│ ├── cityscapes
│ │ ├── annotations
│ │ │ ├── instancesonly_filtered_gtFine_train.json
│ │ │ ├── instancesonly_filtered_gtFine_val.json
│ │ │ ├── instancesonly_filtered_gtFine_test.json
│ │ ├── leftImg8bit
│ │ │ ├── train
│ │ │ ├── val
│ │ ├── gtFine
│ │ │ ├── train
│ │ │ ├── val
7 People in Painting
People in Painting 是一个油画数据集,你可以从 这里, 请注意选择 COCO JSON
格式。然后解压到 data/people_in_painting_v2/
文件夹中。完整的数据集结构如下:
mmdetection
├── configs
├── data
│ ├── people_in_painting_v2
│ │ ├── test
│ │ ├── train
│ │ ├── valid
8 Referring Expression Comprehension
指代性表达式理解的微调和前面一样,也是包括 4 个数据集,在评测数据准备阶段已经全部整理好了,完整的数据集结构如下:
mmdetection
├── configs
├── data
│ ├── coco
│ │ ├── annotations
│ │ │ ├── instances_train2017.json
│ │ │ ├── instances_val2017.json
│ │ │ ├── instances_train2014.json
│ │ ├── train2017
│ │ │ ├── xxx.jpg
│ │ │ ├── ...
│ │ ├── val2017
│ │ │ ├── xxxx.jpg
│ │ │ ├── ...
│ │ ├── train2014
│ │ │ ├── xxx.jpg
│ │ │ ├── ...
│ │ ├── mdetr_annotations
│ │ │ ├── final_refexp_val.json
│ │ │ ├── finetune_refcoco_testA.json
│ │ │ ├── finetune_refcoco_testB.json
│ │ │ ├── finetune_refcoco+_testA.json
│ │ │ ├── finetune_refcoco+_testB.json
│ │ │ ├── finetune_refcocog_test.json
│ │ │ ├── finetune_refcocog_test.json
然后我们需要将其转换为所需的 ODVG 格式,请使用 refcoco2odvg.py 脚本转换,
python tools/dataset_converters/refcoco2odvg.py data/coco/mdetr_annotations
会在 data/coco/mdetr_annotations
中生成新的 4 个 json 文件。 转换后的数据集结构如下:
mmdetection
├── configs
├── data
│ ├── coco
│ │ ├── annotations
│ │ │ ├── instances_train2017.json
│ │ │ ├── instances_val2017.json
│ │ │ ├── instances_train2014.json
│ │ ├── train2017
│ │ │ ├── xxx.jpg
│ │ │ ├── ...
│ │ ├── val2017
│ │ │ ├── xxxx.jpg
│ │ │ ├── ...
│ │ ├── train2014
│ │ │ ├── xxx.jpg
│ │ │ ├── ...
│ │ ├── mdetr_annotations
│ │ │ ├── final_refexp_val.json
│ │ │ ├── finetune_refcoco_testA.json
│ │ │ ├── finetune_refcoco_testB.json
│ │ │ ├── finetune_refcoco+_testA.json
│ │ │ ├── finetune_refcoco+_testB.json
│ │ │ ├── finetune_refcocog_test.json
│ │ │ ├── finetune_refcoco_train_vg.json
│ │ │ ├── finetune_refcoco+_train_vg.json
│ │ │ ├── finetune_refcocog_train_vg.json
│ │ │ ├── finetune_grefcoco_train_vg.json
推理与微调
需要安装额外的依赖包:
cd $MMDETROOT
pip install -r requirements/multimodal.txt
pip install emoji ddd-dataset
pip install git+https://github.com/lvis-dataset/lvis-api.git"
请注意由于 LVIS 第三方库暂时不支持 numpy 1.24,因此请确保您的 numpy 版本符合要求。建议安装 numpy 1.23 版本。
MM Grounding DINO-T 模型权重下载
为了方便演示,您可以提前下载 MM Grounding DINO-T 模型权重到当前路径下
wget load_from = 'https://download.openmmlab.com/mmdetection/v3.0/mm_grounding_dino/grounding_dino_swin-t_pretrain_obj365_goldg_grit9m_v3det/grounding_dino_swin-t_pretrain_obj365_goldg_grit9m_v3det_20231204_095047-b448804b.pth' # noqa
模型的权重和对应的配置详见:
Model | Backbone | Style | COCO mAP | Pre-Train Data | Config | Download |
---|---|---|---|---|---|---|
GDINO-T | Swin-T | Zero-shot | 46.7 | O365 | ||
GDINO-T | Swin-T | Zero-shot | 48.1 | O365,GoldG | ||
GDINO-T | Swin-T | Zero-shot | 48.4 | O365,GoldG,Cap4M | config | model |
MM-GDINO-T | Swin-T | Zero-shot | 48.5(+1.8) | O365 | config | |
MM-GDINO-T | Swin-T | Zero-shot | 50.4(+2.3) | O365,GoldG | config | model | log |
MM-GDINO-T | Swin-T | Zero-shot | 50.5(+2.1) | O365,GoldG,GRIT | config | model | log |
MM-GDINO-T | Swin-T | Zero-shot | 50.6(+2.2) | O365,GoldG,V3Det | config | model | log |
MM-GDINO-T | Swin-T | Zero-shot | 50.4(+2.0) | O365,GoldG,GRIT,V3Det | config | model | log |
MM-GDINO-B | Swin-B | Zero-shot | 52.5 | O365,GoldG,V3Det | config | model | log |
MM-GDINO-B* | Swin-B | - | 59.5 | O365,ALL | config | model | log |
MM-GDINO-L | Swin-L | Zero-shot | 53.0 | O365V2,OpenImageV6,GoldG | config | model | log |
MM-GDINO-L* | Swin-L | - | 60.3 | O365V2,OpenImageV6,ALL | config | model | log |
- 这个*表示模型尚未完全训练。我们将在未来发布最终权重。
- ALL: GoldG,V3det,COCO2017,LVISV1,COCO2014,GRIT,RefCOCO,RefCOCO+,RefCOCOg,gRefCOCO。
推理
在推理前,为了更好的体验不同图片的推理效果,建议您先下载 这些图片 到当前路径下
MM Grounding DINO 支持了闭集目标检测,开放词汇目标检测,Phrase Grounding 和指代性表达式理解 4 种推理方式,下面详细说明。
(1) 闭集目标检测
由于 MM Grounding DINO 是预训练模型,理论上可以应用于任何闭集检测数据集,目前我们支持了常用的 coco/voc/cityscapes/objects365v1/lvis 等,下面以 coco 为例
python demo/image_demo.py images/animals.png \
configs/mm_grounding_dino/grounding_dino_swin-t_pretrain_obj365.py \
--weights grounding_dino_swin-t_pretrain_obj365_goldg_grit9m_v3det_20231204_095047-b448804b.pth \
--texts '$: coco'
会在当前路径下生成 outputs/vis/animals.png
的预测结果,如下图所示
由于鸵鸟并不在 COCO 80 类中, 因此不会检测出来。
需要注意,由于 objects365v1 和 lvis 类别很多,如果直接将类别名全部输入到网络中,会超过 256 个 token 导致模型预测效果极差,此时我们需要通过 --chunked-size
参数进行截断预测, 同时预测时间会比较长。
python demo/image_demo.py images/animals.png \
configs/mm_grounding_dino/grounding_dino_swin-t_pretrain_obj365.py \
--weights grounding_dino_swin-t_pretrain_obj365_goldg_grit9m_v3det_20231204_095047-b448804b.pth \
--texts '$: lvis' --chunked-size 70 \
--palette random
不同的 --chunked-size
会导致不同的预测效果,您可以自行尝试。
(2) 开放词汇目标检测
开放词汇目标检测是指在推理时候,可以输入任意的类别名
python demo/image_demo.py images/animals.png \
configs/mm_grounding_dino/grounding_dino_swin-t_pretrain_obj365.py \
--weights grounding_dino_swin-t_pretrain_obj365_goldg_grit9m_v3det_20231204_095047-b448804b.pth \
--texts 'zebra. giraffe' -c
(3) Phrase Grounding
Phrase Grounding 是指的用户输入一句语言描述,模型自动对其涉及到的名词短语想对应的 bbox 进行检测,有两种用法
这里用到了NLTK 库,首先,寻找NLTK 的文件路径,执行代码:
import nltk
if __name__ == "__main__":
print(nltk.find("."))
如下图:
下载NLTK ,将其放到上面的任意路径。下载链接:https://gitee.com/qwererer2/nltk_data/tree/gh-pages。解压后将packages重新命名为nltk_data,然后将nltk_data移动上面图片中的任意目录。
新建任意脚本,运行下面代码:
from nltk.book import *
出现下图结果则表明没有问题。
- 通过 NLTK 库自动提取名词短语,然后进行检测
python demo/image_demo.py images/apples.jpg \
configs/mm_grounding_dino/grounding_dino_swin-t_pretrain_obj365.py \
--weights grounding_dino_swin-t_pretrain_obj365_goldg_grit9m_v3det_20231204_095047-b448804b.pth \
--texts 'There are many apples here.'
程序内部会自动切分出 many apples
作为名词短语,然后检测出对应物体。不同的输入描述对预测结果影响很大。
- 用户自己指定句子中哪些为名词短语,避免 NLTK 提取错误的情况
python demo/image_demo.py images/fruit.jpg \
configs/mm_grounding_dino/grounding_dino_swin-t_pretrain_obj365.py \
--weights grounding_dino_swin-t_pretrain_obj365_goldg_grit9m_v3det_20231204_095047-b448804b.pth \
--texts 'The picture contains watermelon, flower, and a white bottle.' \
--tokens-positive "[[[21,31]], [[45,59]]]" --pred-score-thr 0.12
21,31 对应的名词短语为 watermelon
,45,59 对应的名词短语为 a white bottle
。
(4) 指代性表达式理解
指代性表达式理解是指的用户输入一句语言描述,模型自动对其涉及到的指代性表达式进行理解, 不需要进行名词短语提取。
python demo/image_demo.py images/apples.jpg \
configs/mm_grounding_dino/grounding_dino_swin-t_pretrain_obj365.py \
--weights grounding_dino_swin-t_pretrain_obj365_goldg_grit9m_v3det_20231204_095047-b448804b.pth \
--texts 'red apple.' \
--tokens-positive -1
评测
我们所提供的评测脚本都是统一的,你只需要提前准备好数据,然后运行相关配置就可以了
(1) Zero-Shot COCO2017 val
# 单卡
python tools/test.py configs/mm_grounding_dino/grounding_dino_swin-t_pretrain_obj365.py \
grounding_dino_swin-t_pretrain_obj365_goldg_grit9m_v3det_20231204_095047-b448804b.pth
# 8 卡
./tools/dist_test.sh configs/mm_grounding_dino/grounding_dino_swin-t_pretrain_obj365.py \
grounding_dino_swin-t_pretrain_obj365_goldg_grit9m_v3det_20231204_095047-b448804b.pth 8
(2) Zero-Shot ODinW13
# 单卡
python tools/test.py configs/mm_grounding_dino/odinw/grounding_dino_swin-t_pretrain_odinw13.py \
grounding_dino_swin-t_pretrain_obj365_goldg_grit9m_v3det_20231204_095047-b448804b.pth
# 8 卡
./tools/dist_test.sh configs/mm_grounding_dino/odinw/grounding_dino_swin-t_pretrain_odinw13.py \
grounding_dino_swin-t_pretrain_obj365_goldg_grit9m_v3det_20231204_095047-b448804b.pth 8
评测数据集结果可视化
为了方便大家对模型预测结果进行可视化和分析,我们支持了评测数据集预测结果可视化,以指代性表达式理解为例用法如下:
python tools/test.py configs/mm_grounding_dino/refcoco/grounding_dino_swin-t_pretrain_zeroshot_refexp \
grounding_dino_swin-t_pretrain_obj365_goldg_grit9m_v3det_20231204_095047-b448804b.pth --work-dir refcoco_result --show-dir save_path
模型在推理过程中会将可视化结果保存到 refcoco_result/{当前时间戳}/save_path
路径下。其余评测数据集可视化只需要替换配置文件即可。
下面展示一些数据集的可视化结果: 左图为 GT,右图为预测结果
- COCO2017 val 结果:
- Flickr30k Entities 结果:
- DOD 结果:
- RefCOCO val 结果:
- RefCOCO testA 结果:
- gRefCOCO val 结果:
模型训练
如果想复现我们的结果,你可以在准备好数据集后,直接通过如下命令进行训练
# 单机 8 卡训练仅包括 obj365v1 数据集
./tools/dist_train.sh configs/mm_grounding_dino/grounding_dino_swin-t_pretrain_obj365.py 8
# 单机 8 卡训练包括 obj365v1/goldg/grit/v3det 数据集,其余数据集类似
./tools/dist_train.sh configs/mm_grounding_dino/grounding_dino_swin-t_pretrain_obj365_goldg_grit9m_v3det.py 8
多机训练的用法请参考 train.md。MM-Grounding-DINO T 模型默认采用的是 32 张 3090Ti,如果你的总 bs 数不是 32x4=128,那么你需要手动的线性调整学习率。
预训练自定义格式说明
为了统一不同数据集的预训练格式,我们参考 Open-GroundingDino 所设计的格式。具体来说分成 2 种格式
(1) 目标检测数据格式 OD
{"filename": "obj365_train_000000734304.jpg",
"height": 512,
"width": 769,
"detection": {
"instances": [
{"bbox": [109.4768676992, 346.0190429696, 135.1918335098, 365.3641967616], "label": 2, "category": "chair"},
{"bbox": [58.612365705900004, 323.2281494016, 242.6005859067, 451.4166870016], "label": 8, "category": "car"}
]
}
}
label字典中所对应的数值需要和相应的 label_map 一致。 instances 列表中的每一项都对应一个 bbox (x1y1x2y2 格式)。
(2) phrase grounding 数据格式 VG
{"filename": "2405116.jpg",
"height": 375,
"width": 500,
"grounding":
{"caption": "Two surfers walking down the shore. sand on the beach.",
"regions": [
{"bbox": [206, 156, 282, 248], "phrase": "Two surfers", "tokens_positive": [[0, 3], [4, 11]]},
{"bbox": [303, 338, 443, 343], "phrase": "sand", "tokens_positive": [[36, 40]]},
{"bbox": [[327, 223, 421, 282], [300, 200, 400, 210]], "phrase": "beach", "tokens_positive": [[48, 53]]}
]
}
tokens_positive 表示当前 phrase 在 caption 中的字符位置。
自定义数据集微调训练案例
为了方便用户针对自定义数据集进行下游微调,我们特意提供了以简单的 cat 数据集为例的微调训练案例。
1 数据准备
cd mmdetection
wget https://download.openmmlab.com/mmyolo/data/cat_dataset.zip
unzip cat_dataset.zip -d data/cat/
cat 数据集是一个单类别数据集,包含 144 张图片,已经转换为 coco 格式。
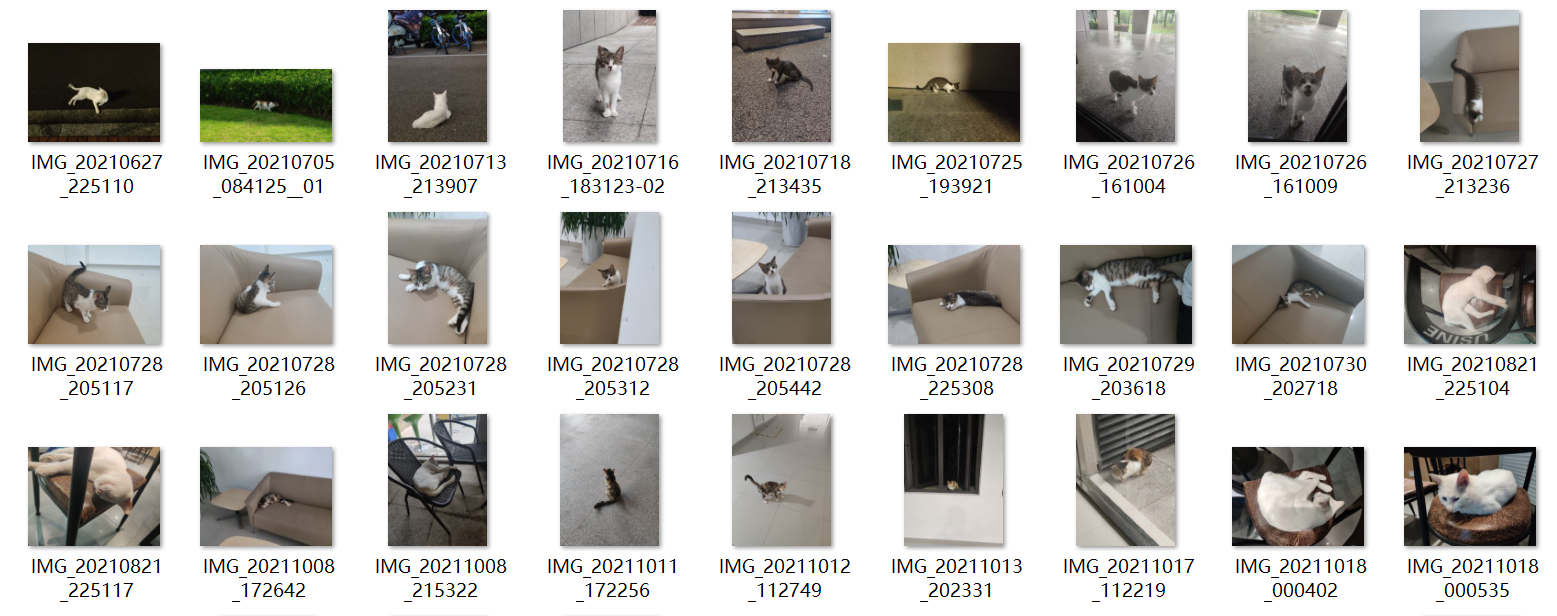
2 配置准备
由于 cat 数据集的简单性和数量较少,我们使用 8 卡训练 20 个 epoch,相应的缩放学习率,不训练语言模型,只训练视觉模型。
详细的配置信息可以在 grounding_dino_swin-t_finetune_8xb4_20e_cat 中找到。
3 可视化和 Zero-Shot 评估
由于 MM Grounding DINO 是一个开放的检测模型,所以即使没有在 cat 数据集上训练,也可以进行检测和评估。
单张图片的可视化结果如下:
cd mmdetection
python demo/image_demo.py data/cat/images/IMG_20211205_120756.jpg configs/mm_grounding_dino/grounding_dino_swin-t_finetune_8xb4_20e_cat.py --weights grounding_dino_swin-t_pretrain_obj365_goldg_grit9m_v3det_20231204_095047-b448804b.pth --texts cat.
测试集上的 Zero-Shot 评估结果如下:
python tools/test.py configs/mm_grounding_dino/grounding_dino_swin-t_finetune_8xb4_20e_cat.py grounding_dino_swin-t_pretrain_obj365_goldg_grit9m_v3det_20231204_095047-b448804b.pth
Average Precision (AP) @[ IoU=0.50:0.95 | area= all | maxDets=100 ] = 0.881
Average Precision (AP) @[ IoU=0.50 | area= all | maxDets=1000 ] = 1.000
Average Precision (AP) @[ IoU=0.75 | area= all | maxDets=1000 ] = 0.929
Average Precision (AP) @[ IoU=0.50:0.95 | area= small | maxDets=1000 ] = -1.000
Average Precision (AP) @[ IoU=0.50:0.95 | area=medium | maxDets=1000 ] = -1.000
Average Precision (AP) @[ IoU=0.50:0.95 | area= large | maxDets=1000 ] = 0.881
Average Recall (AR) @[ IoU=0.50:0.95 | area= all | maxDets=100 ] = 0.913
Average Recall (AR) @[ IoU=0.50:0.95 | area= all | maxDets=300 ] = 0.913
Average Recall (AR) @[ IoU=0.50:0.95 | area= all | maxDets=1000 ] = 0.913
Average Recall (AR) @[ IoU=0.50:0.95 | area= small | maxDets=1000 ] = -1.000
Average Recall (AR) @[ IoU=0.50:0.95 | area=medium | maxDets=1000 ] = -1.000
Average Recall (AR) @[ IoU=0.50:0.95 | area= large | maxDets=1000 ] = 0.913
4 模型训练
./tools/dist_train.sh configs/mm_grounding_dino/grounding_dino_swin-t_finetune_8xb4_20e_cat.py 8 --work-dir cat_work_dir
模型将会保存性能最佳的模型。在第 16 epoch 时候达到最佳,性能如下所示:
Average Precision (AP) @[ IoU=0.50:0.95 | area= all | maxDets=100 ] = 0.901
Average Precision (AP) @[ IoU=0.50 | area= all | maxDets=1000 ] = 1.000
Average Precision (AP) @[ IoU=0.75 | area= all | maxDets=1000 ] = 0.930
Average Precision (AP) @[ IoU=0.50:0.95 | area= small | maxDets=1000 ] = -1.000
Average Precision (AP) @[ IoU=0.50:0.95 | area=medium | maxDets=1000 ] = -1.000
Average Precision (AP) @[ IoU=0.50:0.95 | area= large | maxDets=1000 ] = 0.901
Average Recall (AR) @[ IoU=0.50:0.95 | area= all | maxDets=100 ] = 0.967
Average Recall (AR) @[ IoU=0.50:0.95 | area= all | maxDets=300 ] = 0.967
Average Recall (AR) @[ IoU=0.50:0.95 | area= all | maxDets=1000 ] = 0.967
Average Recall (AR) @[ IoU=0.50:0.95 | area= small | maxDets=1000 ] = -1.000
Average Recall (AR) @[ IoU=0.50:0.95 | area=medium | maxDets=1000 ] = -1.000
Average Recall (AR) @[ IoU=0.50:0.95 | area= large | maxDets=1000 ] = 0.967
我们可以发现,经过微调训练后,cat 数据集的训练性能从 88.1 提升到了 90.1。同时由于数据集比较小,评估指标波动比较大。
模型自训练伪标签迭代生成和优化 pipeline
为了方便用户从头构建自己的数据集或者希望利用模型推理能力进行自举式伪标签迭代生成和优化,不断修改伪标签来提升模型性能,我们特意提供了相关的 pipeline。
由于我们定义了两种数据格式,为了演示我们也将分别进行说明。
1 目标检测格式
此处我们依然采用上述的 cat 数据集为例,假设我们目前只有一系列图片和预定义的类别,并不存在标注。
- 生成初始 odvg 格式文件
import os
import cv2
import json
import jsonlines
data_root = 'data/cat'
images_path = os.path.join(data_root, 'images')
out_path = os.path.join(data_root, 'cat_train_od.json')
metas = []
for files in os.listdir(images_path):
img = cv2.imread(os.path.join(images_path, files))
height, width, _ = img.shape
metas.append({"filename": files, "height": height, "width": width})
with jsonlines.open(out_path, mode='w') as writer:
writer.write_all(metas)
# 生成 label_map.json,由于只有一个类别,所以只需要写一个 cat 即可
label_map_path = os.path.join(data_root, 'cat_label_map.json')
with open(label_map_path, 'w') as f:
json.dump({'0': 'cat'}, f)
会在 data/cat
目录下生成 cat_train_od.json
和 cat_label_map.json
两个文件。
- 使用预训练模型进行推理,并保存结果
我们提供了直接可用的 配置, 如果你是其他数据集可以参考这个配置进行修改。
python tools/test.py configs/mm_grounding_dino/grounding_dino_swin-t_pretrain_pseudo-labeling_cat.py \
grounding_dino_swin-t_pretrain_obj365_goldg_grit9m_v3det_20231204_095047-b448804b.pth
会在 data/cat
目录下新生成 cat_train_od_v1.json
文件,你可以手动打开确认或者使用 脚本 可视化效果
python tools/analysis_tools/browse_grounding_raw.py data/cat/ cat_train_od_v1.json images --label-map-file cat_label_map.json -o your_output_dir --not-show
会在 your_output_dir 目录下生成可视化结果
- 继续训练提高性能
在得到伪标签后,你可以混合一些预训练数据联合进行继续预训练,提升模型在当前数据集上的性能,然后重新运行 2 步骤,得到更准确的伪标签,如此循环迭代即可。
2 Phrase Grounding 格式
- 生成初始 odvg 格式文件
Phrase Grounding 的自举流程要求初始时候提供每张图片对应的 caption 和提前切割好的 phrase 信息。以 flickr30k entities 图片为例,生成的典型的文件应该如下所示:
[
{"filename": "3028766968.jpg",
"height": 375,
"width": 500,
"grounding":
{"caption": "Man with a black shirt on sit behind a desk sorting threw a giant stack of people work with a smirk on his face .",
"regions": [
{"bbox": [0, 0, 1, 1], "phrase": "a giant stack of people", "tokens_positive": [[58, 81]]},
{"bbox": [0, 0, 1, 1], "phrase": "a black shirt", "tokens_positive": [[9, 22]]},
{"bbox": [0, 0, 1, 1], "phrase": "a desk", "tokens_positive": [[37, 43]]},
{"bbox": [0, 0, 1, 1], "phrase": "his face", "tokens_positive": [[103, 111]]},
{"bbox": [0, 0, 1, 1], "phrase": "Man", "tokens_positive": [[0, 3]]}]}}
{"filename": "6944134083.jpg",
"height": 319,
"width": 500,
"grounding":
{"caption": "Two men are competing in a horse race .",
"regions": [
{"bbox": [0, 0, 1, 1], "phrase": "Two men", "tokens_positive": [[0, 7]]}]}}
]
初始时候 bbox 必须要设置为 [0, 0, 1, 1]
,因为这能确保程序正常运行,但是 bbox 的值并不会被使用。
{"filename": "3028766968.jpg", "height": 375, "width": 500, "grounding": {"caption": "Man with a black shirt on sit behind a desk sorting threw a giant stack of people work with a smirk on his face .", "regions": [{"bbox": [0, 0, 1, 1], "phrase": "a giant stack of people", "tokens_positive": [[58, 81]]}, {"bbox": [0, 0, 1, 1], "phrase": "a black shirt", "tokens_positive": [[9, 22]]}, {"bbox": [0, 0, 1, 1], "phrase": "a desk", "tokens_positive": [[37, 43]]}, {"bbox": [0, 0, 1, 1], "phrase": "his face", "tokens_positive": [[103, 111]]}, {"bbox": [0, 0, 1, 1], "phrase": "Man", "tokens_positive": [[0, 3]]}]}}
{"filename": "6944134083.jpg", "height": 319, "width": 500, "grounding": {"caption": "Two men are competing in a horse race .", "regions": [{"bbox": [0, 0, 1, 1], "phrase": "Two men", "tokens_positive": [[0, 7]]}]}}
你可直接复制上面的文本,并假设将文本内容粘贴到命名为 flickr_simple_train_vg.json
文件中,并放置于提前准备好的 data/flickr30k_entities
数据集目录下,具体见数据准备文档。
- 使用预训练模型进行推理,并保存结果
我们提供了直接可用的 配置, 如果你是其他数据集可以参考这个配置进行修改。
python tools/test.py configs/mm_grounding_dino/grounding_dino_swin-t_pretrain_pseudo-labeling_flickr30k.py \
grounding_dino_swin-t_pretrain_obj365_goldg_grit9m_v3det_20231204_095047-b448804b.pth
会在 data/flickr30k_entities
目录下新生成 flickr_simple_train_vg_v1.json
文件,你可以手动打开确认或者使用 脚本 可视化效果
python tools/analysis_tools/browse_grounding_raw.py data/flickr30k_entities/ flickr_simple_train_vg_v1.json flickr30k_images -o your_output_dir --not-show
会在 your_output_dir
目录下生成可视化结果,如下图所示:
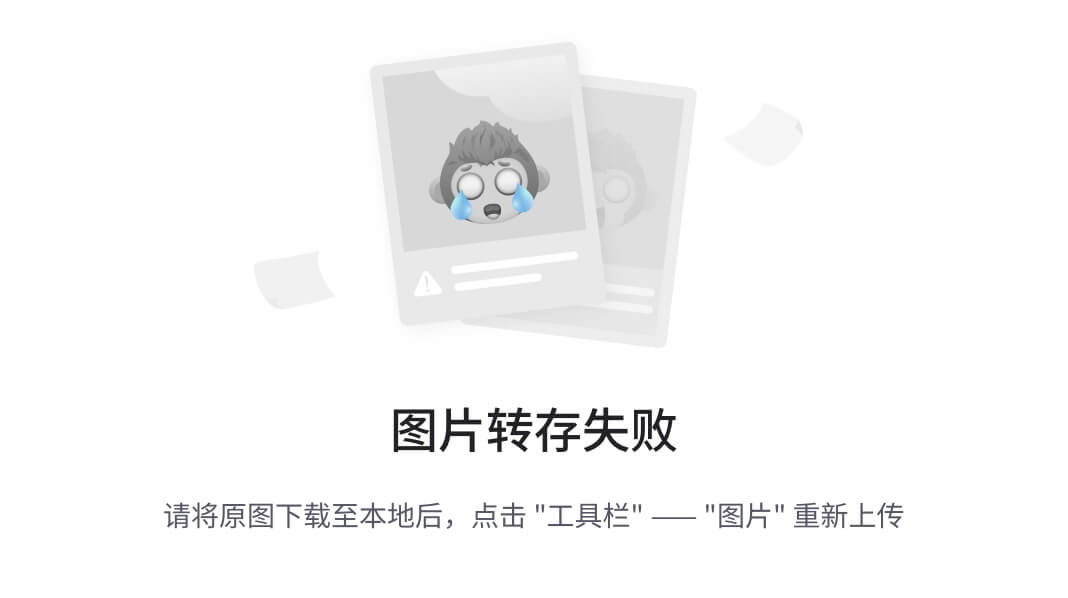
- 继续训练提高性能
在得到伪标签后,你可以混合一些预训练数据联合进行继续预训练,提升模型在当前数据集上的性能,然后重新运行 2 步骤,得到更准确的伪标签,如此循环迭代即可。